MPRG iSearch | Information Search, Ecological and Active Learning Research with Children
Head: Azzurra Ruggeri
1. Emergence and Developmental Trajectory of Active and Ecological Learning
2. Mechanisms of Developmental Change
3. The Role of Active Learning in Navigating Social Environments
4. Interventions to Boost Learning
The great pioneer of computer science, Alan Turing, famously believed that to build a general artificial intelligence, one must create a machine that can learn like a child. However, this dream seems to be still quite out of reach. A child is indeed the only known system that develops into an intelligent agent through playful exploration. However, to this day, we do not know much about how children explore or why—what motivates them, what drives their specific exploratory actions and patterns, how active exploration and question asking support their learning, or to what extent this behavior is purposeful and goal directed. We do not even know exactly whether and in what ways children’s exploratory and learning behavior differs from that of adults.
Indeed, despite their capital importance, there has not been much work trying to answer these questions. Most of the developmental research in the last decade has been fueled by the idea that children are curious explorers, and by the metaphor of the “child as a scientist,” which is intriguing and admittedly very powerful but also has its limits, being a little too vague on the whys and hows, and a little shallow on the mechanisms and the processes, which are often investigated only superficially. The iSearch research program addresses these questions by focusing precisely on the mechanisms underpinning individual differences and developmental changes to work out how children become adults by exploring and learning in active, meaningful, self-directed ways. By bringing together methods and insights from developmental and cognitive psychology, philosophy, education, information theory, and computational modeling, our research sheds light on the cognitive, social, and cultural mechanisms underlying children’s ability to learn and face uncertain—less or more expected—future challenges, from adapting to a dynamic job market, to studying a new subject, to facing a pandemic.
In particular, from this unique and highly multidisciplinary perspective, we can (1) objectively measure and quantify developmental differences in active and ecological learning, tracing their emergence, and (2) develop and compare alternative models of children’s and adults’ behavior, capturing the individual differences and the mechanisms driving and constraining developmental changes. This more computational groundwork generates insights, paradigms, and theoretical frameworks that (3) open new perspectives on the study of children’s learning in social and digital environments, and (4) can inform the development of more effective interventions to support children’s learning potential in classroom settings and beyond.
In the last 6 years, iSearch has tested over 5,000 children and adult participants by conducting experiments in the lab and, more recently, online, and establishing long-term collaborations with public and private kindergartens and schools, museums such as the Natural History Museum and the Labyrinth Children’s Museum (see Figure 1), and the Berlin Zoo. Also, we have tested over 600 children in collaboration with colleagues from universities and partner institutions in the United States, Cuba, Italy, India, and Egypt.
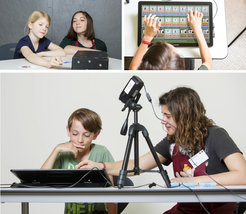
In what follows, we give an overview of these four research themes, highlighting iSearch’s scientific achievements of the last 6 years, as well as current and future directions.
1. Emergence and Developmental Trajectory of Active and Ecological Learning
The work of iSearch investigates theoretically and empirically how children actively seek information in their physical and social environments to test and dynamically revise their hypotheses and theories. We explore the effectiveness of children’s information-search and hypothesis-testing strategies, such as question asking and active exploration, tracing their emergence and developmental trajectory. Crucially, the effectiveness of such active learning strategies cannot be measured in absolute terms. Instead, their effectiveness depends on the characteristics of the task at hand and the available resources, as well as on the learners’ prior knowledge and expectations. In this sense, to maximize learning effectiveness, it is crucial to be able to adapt one’s active learning strategies to the current situation—an ability we refer to as ecological active learning (Ruggeri, 2022). For example, the quality of a question has been traditionally tied to whether it is hypothesis scanning or constraint seeking (Mosher & Hornsby, 1966). Hypothesis-scanning questions are tentative solutions, that is, single objects or hypotheses that are tested directly (e.g., “Was he late because he woke up late?”). Constraint-seeking questions instead aim to reduce the space of possible hypotheses by targeting categories or testing features shared by several different hypotheses (“Was he late because he could not find something?”). Constraint-seeking questions have been generally considered more informative than hypothesis-scanning questions, because they allow the question asker to rule out multiple hypotheses at once, thus reducing the number of questions needed to identify the solution. However, this is not always the case. Indeed, this qualitative distinction does not necessarily reflect the questions’ actual informativeness, as can be formally measured, for example, by calculating their expected information gain (EIG), that is, their expected reduction in uncertainty (Lindley, 1956; Shannon, 1948).
From this more quantitative, computational perspective, we were able to provide the first evidence demonstrating that ecological active learning emerges early in development. Seven- and 10-year-olds generate different types of questions depending on the likelihood distribution of the hypotheses under consideration, in order to maximize the questions’ informativeness (Ruggeri & Lombrozo, 2015). Also, by age seven, children are as sensitive as adults to the causal sparsity (i.e., the number of variables that are likely to impact an outcome) of an unfamiliar causal system, and use this information to tailor their testing strategies (Bramley et al., 2022; for the computational framework and results with adults, see Coenen et al., 2019). Also, this approach allowed us to capture how children’s active physical learning is as effective and goal-targeted as adults’ (Bramley & Ruggeri, 2022). In more recent work, we extended this investigation to explore younger children’s ecological active learning abilities and found that at 5 years old, children already rely on different types of questions depending on the likelihood of the available hypotheses (Ruggeri, Sim, & Xu, 2017). By implementing a nonverbal version of this same paradigm, we were able to demonstrate that even 3- and 4-year-olds are already able to adapt their exploratory strategies to the statistical structure of a given task (Ruggeri, Swaboda, Sim, & Gopnik, 2019).
2. Mechanisms of Developmental Change
The work presented above illustrates how the computational framework we adopted allowed us to measure and capture the developmental trajectory of active and ecological learning. However, computational methods also enable the studying of developmental changes from the process level, a deeper and richer perspective. By developing and comparing models of children’s and adults’ behavior, this approach makes it possible to (a) deconstruct and disentangle the mechanisms underlying developmental change, (b) identify the contributions of individual, age-related, and environmental factors to active learning, and (c) capture what drives children’s curiosity and persistence.
a) The mechanisms underlying developmental differences. Foraging for food, developing new medicines, and learning complex games are search problems with vast numbers of possible actions. Under real-world time or resource constraints, exhaustive exploration is impossible and optimal solutions are generally unobtainable. How do children and adults learn what actions to take when not all outcomes can be explored, balancing exploration and exploitation? On the one hand, we want to explore unfamiliar options that can provide useful information for future decisions, but they may result in poor immediate rewards. On the other hand, we want to exploit options that we know are highly rewarding, but this often implies giving up on learning about unexplored options. A key finding in the psychological literature is that children tend to try out more options than adults, and this has been interpreted as evidence for higher levels of random exploration. They would initially sample very randomly across a large set of possibilities before eventually focusing on a smaller subset. This high temperature parameter is then expected to “cool off” with age, leading to lower levels of random exploration in late childhood and adulthood. However, there are other potential explanations for developmental differences in search. Developmental changes in the variability of sampling could also be explained by changes in directed exploration. In this sense, children’s search appears more scattered, but only because they are more likely to seek highly uncertain options in order to gain more information and reduce uncertainty about the environment, and learn. Rather than seeing development as a change in how children explore, we could explain developmental differences in sampling as a refinement of cognitive processes, leading to a broader generalization. That is, development might be associated with a more fine-grained ability to generalize beyond observed outcomes, thereby guiding search by forming inductive beliefs about novel options.
We tested the individual contribution of these three, not mutually exclusive mechanisms by developing a spatial search task in which 7- to 11-year-old children and adults were presented with a grid. A single tile was initially revealed, and participants were given a limited number of clicks to acquire as many points as possible. Rewards were spatially correlated, with nearby tiles having similar rewards, where the patches were identifiable by color. This spatial structure of the environment provided traction for generalization, which could be used to guide search and could support this generalization more, as in smooth environments, or less, as in rough environments.
We found that adults gained higher rewards than children and exploited more strongly; that is, they were more likely to re-click, whereas children sampled more unique options, thereby gaining lower rewards but exploring the environment more extensively. Using a computational model with parameters directly corresponding to the three hypothesized mechanisms of developmental change, we found that children, compared to adults, generalized less from observed to unobserved tiles and relied on uncertainty-directed exploration more. We did not, however, find differences in random sampling. Overall, our results shed new light on the developmental trajectories in generalization and exploration, casting children not as merely prone to more random sampling behavior but as directed explorers who are hungry for information in their environment (Schulz et al., 2019). Using the same paradigm, we extended this developmental investigation to include 4- to 7-year-old children and found that although younger children tended to be more random in their search compared to older children and adults, even 4-year-olds showed uncertainty-directed exploratory patterns (Meder et al., 2021).
b) Individual, task-related, and environmental differences in active learning. Existing research on active learning has focused on identifying key developmental differences in the efficiency and adaptiveness of children’s search. This research has highlighted three important sources of developmental change: an increasing ability to recognize and exploit the abstract, hierarchical structure of the hypothesis space (Chai et al., 2023; Jones et al., 2021; Ruggeri & Feufel, 2015; Ruggeri et al., 2021), the ability to adapt one’s search strategy based on task characteristics (Ruggeri & Lombrozo, 2015; Ruggeri et al., 2019), and a growing ability to implement efficient rules to stop searching for more information (Ruggeri et al., 2016). However, we do not yet understand why these changes occur or what factors underlie the observed developmental trajectories. More specifically, we do not know what task-related, environmental, and individual factors drive developmental changes in active learning, how they interact with each other, or how their relative importance changes with age.
To answer these critical questions, in a cross-cultural collaboration with partners in Cuba, Egypt, and India (see Figure 2, right), we are currently writing up a complex Structural Equation Modeling exploratory analysis aiming to identify and map the factors contributing to active learning performance, beyond the broad developmental differences captured in previous research. On the one hand, the project aims to measure different aspects of active learning (e.g., effectiveness, adaptiveness, speed, accuracy) on a wide range of tasks (e.g., question asking, question evaluation, spatial search) to comprehensively assess 6- to 11-year-old children’s active learning performance (see Figure 2, left). On the other hand, we will systematically examine the cognitive, social, motivational, cultural, and socioeconomic factors impacting and contributing to active learning performance, to identify the sources of the developmental differences and interpret the individual differences observed. In this sense, the cross-cultural design allows us not only to examine a broader range of cultural differences (e.g., type of education) and to assess the robustness of our methods, but also to generalize our results to populations not usually represented in psychological research.
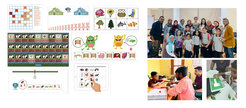
c) What drives children’s curiosity. The work presented in this section shows that children engage in meaningful and effective exploration, but it does not address the question of whether, in general, the opportunity to gain information is enough to motivate children’s search. This is one of the greatest challenges for artificial intelligence—how to behave adaptively in scenarios with sparse or no rewards. Psychologists, computer scientists, and roboticists suggest that “curiosity-based” systems may do better than standard reinforcement learning methods. These systems have in common the idea that quantitative increases in information gain are themselves rewarding and motivate actions, and they are often explicitly inspired by the curiosity-based exploration of young children. It does indeed seem crystal clear that young children are curious and that this contributes to their impressive learning abilities. However, thus far there has been no work investigating what motivates children to explore and learn in the absence of external rewards. In a recent paper (Ruggeri et al., 2023), we investigated whether and to what extent the opportunity to gain information motivates young children’s exploratory actions, that is, whether expected information gain serves as an intrinsic reward, strong enough to drive exploration and learning.
In three studies, we measured 24- to 56-month-old children’s persistence in a game in which they had to search for a cartoon animal hidden behind a series of closed doors. Crucially, we manipulated the degree of uncertainty about which specific animal participants were searching for; that is, either we told children which animal they were searching for (e.g., the lion), or we told them that it could be one of eight different animals—so that finding the hidden animal gave children information about its identity, in addition to discovering its location (see Figure 3, top). Although the hidden animal was never revealed, regardless of the time children spent searching, our results indicate that children were more persistent in their search in the eight-animals condition (in orange in Figure 3c), when there was higher uncertainty as to which animal they would find, and therefore more information to be gained: They opened more doors on average and searched for a longer time, and more of them persevered and kept on opening doors until the end of the game (see Figure 3, bottom). These results indicate that, in the absence of any rewards, toddlers and preschoolers’ search is motivated by the expected informativeness of the actions that can be performed, highlighting how important it is for research on artificial intelligence to invest in curiosity-driven algorithms.
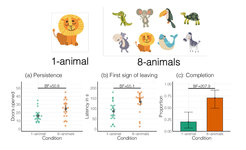
Original image licensed under CC-BY 4.0
3. The Role of Active Learning in Navigating Social Environments
The developmental and computational work on active learning we did in past years generated insights and offered new methods that can enrich and deepen our understanding of children’s social learning. Effective learning requires the ability to identify from whom to learn and whom to ask for help. Despite much prior work on children’s evaluation of informants in pedagogical contexts and their ability to selectively learn from more informative teachers, little is known about children’s sensitivity to how potential teachers come to know what they know. Do children consider the process of others’ learning to decide from whom to learn? By choosing to seek help from other individuals who are better learners themselves, children can maximize opportunities for learning how to learn. Indeed, we found that adult-like meaningful and selective inferences based on other people’s ability to search effectively emerge around age five (De Simone & Ruggeri, 2021). Along these lines, in a recent series of studies we showed that even young children prefer to learn from successful active learners and choose to learn from such learners selectively depending on the context (Bridgers et al., 2023). These results are interesting, in two different ways: First, they suggest that already preschoolers take into account how an agent reached a certain outcome, beyond the observed effectiveness of an agent’s actions. However, in a recent paper (Törok et al., 2023) we showed that until age eight children struggle to infer competence from more implicit cues, such as expected strategy efficiency, and that success and observed strategy effectiveness (for example the duration of exploration), sometimes erroneously, remains a powerful cue for competence throughout childhood and even in adulthood. Second, they suggest that children may be able to disentangle, to a certain extent, between someone who knows things—an expert—and someone who is not knowledgeable but is competent in learning about new things, for example, someone who is good at asking questions (De Simone & Ruggeri, 2022).
4. Interventions to Boost Learning
How can we boost children’s learning success in classroom settings and beyond? One way to do this is to develop interventions that enhance children’s learning performance by supporting the effectiveness of their active learning strategies, for example, helping them ask more informative questions. Previous attempts to improve children’s question-asking strategies have achieved only moderate success. Most children did not improve their performance over time, and the modest training benefits, when present, did not generalize to other sets of stimuli or domains and were no longer apparent just a few days later. However, our work shows that it is indeed possible to support children’s question-asking performance even without extensive training (see Ruggeri et al., 2021). For example, we found that prompting children to explain previously observed sets of causal data helped 6- and 7-year-olds, but not younger children, ask more effective questions (Ruggeri et al., 2019). Improving children’s active inquiry skills at an early age has the potential to accelerate the development of their general information-search strategies and problem-solving skills, boosting their later independent learning.
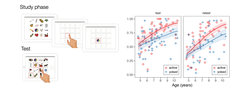
Another way to boost children’s learning success is to design learning environments that enhance their performance. For example, in a series of computational modeling studies, Ruggeri, Markant, Gureckis, Bretzke, and Xu (2019) found that having the opportunity to actively control the study experience—for example, to decide what to study, when, and for how long (see Figure 4, left)—leads to enhanced learning in 5- to 11-year-old children, compared to conditions that lack this control (see Figure 4, right). We recently replicated these results with a population of children affected by autism (Fantasia et al., 2020). In a recently published work, we also showed that a spatial representation of a search task supports 4- to 7-year-old children’s information-search strategies, relative to their performance in a question-asking game (Swaboda et al., 2022). These results also demonstrate that efficient search strategies emerge much earlier than previously assumed, highlighting the importance of developing age-appropriate paradigms that capture children’s early competence to gain a more comprehensive picture of their emerging information-search abilities.
Conclusion
To conclude, the iSearch research program inaugurates a new perspective, ecological active learning, that challenges a simple data-driven view of knowledge acquisition and change, in which children’s learning is only a function of their casual observations and the teaching from others. Instead, thanks to the novel integration of developmental and computational methods, our work sheds new light on the mechanisms underlying and driving developmental trajectories in exploration, casting children as motivated and curious learners who are hungry for information in their environment, but also sensitive, selective, effective, and—above all—adaptive.
Key Rferences
Bramley, N., Jones, A., Gureckis, T. M., Ruggeri, A. (in press) Changing many things at once sometimes makes for a good experiment, and children know that. Psychonomic Bulletin and Review.
Bridgers, S., De Simone, C., Gweon, H., & Ruggeri, A. (2023). How you learned matters: The process by which others learn informs whom children ask for help. Child Development.
Chai, K., Xu, F., Swaboda, N., & Ruggeri, A. (2023) Sources of inefficiency in preschoolers’ information search. Frontiers in Psychology. doi: 10.3389/fpsyg.2022.1080755
Fantasia, V., Markant, D., Perri, N., Valeri, G., & Ruggeri, A. (2020). Benefits of active control of study in autistic children. Autism, 24(8), 1995–2007. https://doi.org/10.1177/1362361320931244
Jones, A., Markant, D., Gopnik, A., Pachur, T., & Ruggeri, A. (2021). Developmental differences in active causal learning across preschool age reveal different hypothesis-space structures. Developmental Psychology.
Lindley, D. V. (1956). On a measure of the information provided by an experiment. Annals of Mathematical Statistics, 27, 986–1005. https://doi: 10.1214/aoms/1177728069
Mosher, F. A., & Hornsby, J. R. (1966). On asking questions. In J. S. Bruner, R. R. Olver, T. M. Greenfield, J. R. Hornsby, H. J. Kenney, M. Maccoby, et al. (Eds.), Studies in cognitive growth, pp. 86–102. New York: Wiley.
Ruggeri, A., Stanciu, O., Pelz, M., Gopnik, A., & Schulz, E. (2023). Toddlers and preschoolers search longer when there is more information to be gained. Developmental Science.
Ruggeri, A., Walker, C., Lombrozo, T. & Gopnik, A. (2021). Strategic use of questions to solve a causal inference task in early childhood. Frontiers in Psychology, 11, Article 586819. https://doi.org/10.3389/fpsyg.2020.586819
Shannon, C. E. (1948). A mathematical theory of communication. The Bell System Technical Journal, 27, 379–423, 623–656.