Director Emeritus Gerd Gigerenzer
Psychological Heuristics for a Safer Financial System: A Collaboration With the Bank of England
Adaptive Heuristics and Machine Learning
Psychological AI
Recency: One Data Point Can Beat Big Data
Predicting the Flu
Human Intelligence ≠ Machine Intelligence
Do Children have Bayesian Intuitions?
Misleading Statistics in the Media
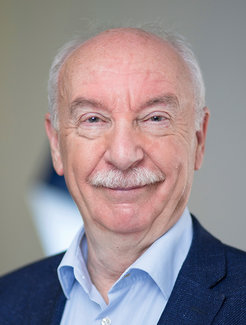
The ABC Research Group, established at the MPI for Psychological Research in Munich in 1995, moved to the MPI for Human Development in Berlin in 1997 and ended its local activities on 30 September 2017. Gerd Gigerenzer has continued as Director of the Harding Center for Risk Literacy, which was first located at the MPI for Human Development and moved to the University of Potsdam in 2020. Research from the Harding Center is not included in this report.
Psychological Heuristics for a Safer Financial System: A Collaboration With the Bank of England
In recent years, the financial system has become increasingly complex. Both the private sector and public authorities have tended to meet this complexity head-on, be it through increasingly complex modeling and risk management strategies or ever-lengthening regulatory rulebooks. Yet this helped neither to predict nor to prevent the global financial crisis of 2007–2008. In collaborative work with the Bank of England, we analyzed adaptive heuristics that are transparent and can predict bank failure better than standard complex models.
Distinguishing between risk and uncertainty, Aikman et al. (2021) draw on the psychological literature on heuristics to consider whether and when simpler approaches may outperform more complex methods for modeling and regulating the financial system. We found that (a) simple methods can dominate more complex modeling approaches for calculating banks’ capital requirements, especially when data are limited or underlying risks are fat-tailed; (b) simple indicators often outperformed more complex metrics in predicting individual bank failure during the global financial crisis; and (c) when combining different indicators to predict bank failure, simple and easy-to-communicate “fast-and-frugal” decision trees can perform comparably to standard but more information-intensive regressions. Taken together, our analyses suggest that because financial systems are better characterized by uncertainty than by risk, simpler approaches to modeling and regulating financial systems can usefully complement more complex approaches and ultimately contribute to a safer financial system.
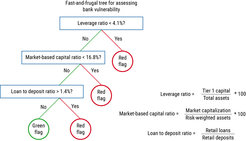
Figure 1. A fast-and-frugal tree for assessing bank vulnerability. The tree is based on a combination of expert judgment (to identify the relevant variables) and data (to set the thresholds). It predicts bank failure as accurately as or more accurately than logistic regression models and is, moreover, transparent and easy to apply. Image: MPI for Human Development
Image: MPI for Human Development
Adapted from Gigerenzer et al. (2021)
Original image licensed under CC BY-4.0
Key Reference
Adaptive Heuristics and Machine Learning
As the research with the Bank of England illustrates, fast-and-frugal trees and other heuristics can deal with situations of radical uncertainty (as opposed to calculable risk), while being transparent and easy to understand and teach. For a central bank, transparency is essential because it makes gaming easier to detect.
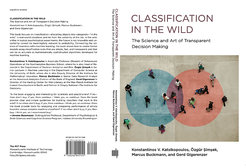
In the book Classification in the Wild (Katsikopoulos et al., 2020), we address the general questions of the relationship between psychological heuristics and machine learning. The two classes of heuristics we analyze are ecologically rational for different types of environments. First, fast-and-frugal trees exploit situations with dominant cues (variables), that is, where the (beta) weights of cues are steeply skewed, for example exponentially decreasing. Second, we look at tallying heuristics, which are adapted to situations where the weights of the cues are more equal. The book analyzes the conditions under which simple heuristics predict as accurately as random forests and other machine learning techniques, in both out-of-sample and out-of-population prediction. It also provides a hands-on introduction to the design of fast-and-frugal trees and tallying rules, such as the balancing of the false-positive rate with the miss rate. Classification in the Wild will also be translated into Chinese and published by East Babel.
Psychological AI
The fast-and-frugal tree for identifying volatile banks illustrates how the program of psychological AI uses the structure of heuristics humans rely on for designing predictive algorithms. In general, psychological AI applies insights from psychology to design computer algorithms. Its core domain is decision making under uncertainty, that is, ill-defined situations that can change in unexpected ways rather than well-defined, stable problems. Psychological theories about heuristic processes under uncertainty can provide possible insights. The following case study shows how recency—the human tendency to rely on the most recent information and ignore base rates—can be built into a simple algorithm that predicts the flu substantially better than Google Flu Trends’ big data algorithms did. This case study provides an existence proof that psychological AI can help design efficient and transparent algorithms.
Recency: One Data Point Can Beat Big Data
Recency is the tendency to attach more importance to recent events than to past ones. In memory research, a recency effect has been observed that occurs when events more recently encountered are remembered best. Recency is sometimes considered a bias in the sense of error, as in the availability bias, but this valuation overlooks that recency, like all psychological phenomena, is neither rational nor irrational in itself. Rather, one needs to assess its ecological rationality. In a stable world, relying on the most recent events only and ignoring the base rates (the data of the past) might indeed be an error. But in an unstable world, where unexpected events happen, relying on recency may well lead to better decisions.
Predicting the Flu
To indicate where and how rapidly the flu was spreading, Google engineers used big data analytics to predict the rate of flu-related doctor visits on a daily or weekly basis. They analyzed 50 million queries submitted to the Google search engine and selected 45 variables from these, which they combined in a secret algorithm dubbed Google Flu Trends (GFT) (Ginsberg et al., 2009). GFT was trained on data from 2003 to 2007 and tested on data from 2007 to 2008. After the swine flu struck in the spring of 2009, however, GFT consistently underpredicted the number of flu-related doctor visits over months (see Figure 2). It had learned that the rate of flu is high in the winter and low in the summer, but the swine flu followed a different rhythm. To improve the algorithm, the engineers opted to make it more complex and increased the number of variables to approximately 160. Additional complexity, however, provided no improvement; the updated version now overestimated the proportion of flu-related doctor visits in 100 out of 108 weeks from August 2011 to September 2013, in some cases overshooting the actual visits by more than 50% (Figure 2). In response, the Google engineers again suspected that their model was too simple and updated it once again in 2013. After yet another update in 2014, GFT was shut down in 2015.
Instead of betting on more data and increasing the complexity of the algorithm, the alternative approach is psychological AI. In fast-changing environments, such as those involving flu viruses, it is rational to decrease complexity and ignore past data. Katsikopoulos et al. (2022) designed the simplest version of the recency heuristic, which implements these principles and relies on a single data point. We programmed recency into a fast-and-frugal algorithm:
Recency heuristic: Predict that this week’s proportion of flu-related doctor visits equals the proportion from the most recent week.
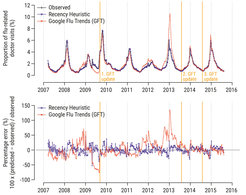
One can intuitively see that this algorithm can adapt quickly to sudden and unexpected events such as the swine flu, whereas big data makes it difficult to change course. We tested the weekly predictions of the recency heuristic from 18 March 2007 to 9 August 2015 (the same period in which GFT made predictions). The overall error was measured by the average absolute difference between the predicted and observed values of flu-related doctor visits divided by the observed value (mean absolute prediction error). For the recency heuristic, the mean error was 9%, compared with 20% for GFT. The advantage of the psychologically inspired algorithm held for every year between 2007 and 2015, and across all three updates of GFT. Here, one data point was more powerful than big data. This case is an existence proof of the benefits of psychological AI.
Image: International Journal of Forecasting
Adapted from Katsikopoulos et al. (2022)
Algorithms that embody recency have also been shown to be superior to complex macroeconomic models in predicting demand (Dosi, Napoletano, Roventini, Stiglitz, & Treibich, 2020), and have predicted future customer purchases, sports events, and health behavior as accurately as random forests and logistic regressions, even though the latter used more data points (Artinger, Kozodoi, von Wangenheim, & Gigerenzer, 2018).
Key References
Human Intelligence ≠ Machine Intelligence
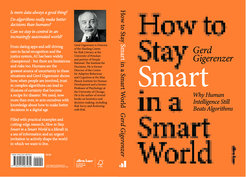
In the book How to Stay Smart in a Smart World (2022), I describe the psychological mechanisms used to keep users addicted to checking their phones and staying on social media platforms, and discuss heuristics such as lateral reading to determine the trustworthiness of websites. A key theme is the differences between human intelligence, intuition, and common sense, and deep artificial neural networks. Common sense is shared knowledge about people and the physical world, enabled by the biological brain. It comprises intuitive psychology, intuitive physics, and intuitive sociality. Unlike deep neural networks, common sense requires only limited experience. Human intelligence has evolved to deal with uncertainty, independent of whether big or small data are available. Complex AI algorithms, in contrast, work best in stable, well-defined situations such as chess and Go, where large amounts of data are available. This stable-world principle helps an understanding of what statistical algorithms are capable of and distinguishes this from commercial hype or techno-religious faith. I also introduce the program of psychological AI, which uses psychological heuristics to make algorithms smart. What AI needs is a fusion of the adaptive heuristics that embody common sense with the power of machine learning.
The book has been translated into Chinese (mainland), complex Chinese (Taiwan), Finnish, German, Italian, Korean, Polish, Russian, and Romanian.
Do children have Bayesian Intuitions?
Can children solve Bayesian problems, given that these pose great difficulties even for most adults? Gigerenzer, Multmeier, Föhring, and Wegwarth (2021) present an ecological framework in which Bayesian intuitions emerge from a match between children’s numerical competencies and external representations of numerosity. Bayesian intuition is defined here as the ability to determine the exact Bayesian posterior probability by minds untutored in probability theory or in Bayes’s rule. As we show, Bayesian intuitions do not require processing of probabilities or Arabic numerals, but basically the ability to count tokens in icon arrays and to understand what to count. A series of experiments demonstrates for the first time that icon arrays elicited Bayesian intuitions in children as young as second-graders for 22% to 32% of all problems; fourth-graders achieved 50% to 60%.
One of the problems children were given is the magic wand problem:
Out of every 20 students of Hogwarts School of Witchcraft, five have a magic wand. Of these five students, four also have a magic hat. Of the other 15 students without a magic wand, 12 also have a magic hat.
Imagine you meet a group of students at Hogwarts School who have a magic hat. How many of them have a magic wand? ___out of ___.
Most surprisingly, icon arrays elicited Bayesian intuitions in children with dyscalculia, a specific learning disorder that has been attributed to genetic causes. These children could solve an impressive 50% of Bayesian problems, a level similar to that of children without dyscalculia. By seventh grade, children solved about two thirds of Bayesian problems with natural frequencies alone, without the additional help of icon arrays. We also identified four non-Bayesian rules. On the basis of these results, we propose a common solution for the phylogenetic, the ontogenetic, and the 1970s puzzles in the Bayesian literature and argue for a revision of how to teach statistical thinking. In accordance with recent work on infants’ numerical abilities, these findings indicate that children have more numerical ability than previously assumed.
Key Reference
Misleading Statistics in the Media
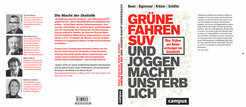
Every month, three colleagues and I select a media report that misrepresents numbers and draws wrong conclusions as the “Unstatistik des Monats” (unstatistik.de), that is, the misleading statistics of the month. In our book Grüne fahre SUV und Joggen macht unsterblich (Greens drive SUVs and jogging makes you immortal), we compiled and edited a selection of these reports. Among the sins journalists commit year after year, either due to lack of understanding or done intentionally to attract attention, are (a) presenting correlations as causes; (b) presenting the effect of medical treatments with relative numbers instead of absolute numbers, which inflates the actual effect; (c) misinterpreting the reference class of a percentage reported; and (d) failing to understand that every test makes two errors and that reporting the hit rate of the newest blood test for cancer or the newest facial recognition system means nothing if the false alarm rate is not reported. We do this work to compensate for the dearth of journalists’ education in statistical thinking and to help the general public see through the misconceptions and learn what critical questions to ask.
The book is currently being translated into Korean, to be published by Onward.
Key Reference