Area 1: Learning Representations of Values and States—Cognitive Maps in Hippocampus, Orbitofrontal Cortex, and Beyond
Project 1.1: Representation Learning of Task States
Project 1.2: Effects of Reward of Neural Representations of Spatial
Project 1.4: Representation Learning Dynamics Underlying Aha-Moments
For decision making and learning to be effective, one must learn internal representations that suitably abstract high-dimensional inputs such that associations can be learned based on a much more conceptual level than, say, individual pixels of a scene. Even a simple task such as buying an apple in a grocery shop can be intractable if one does not have a reductive transformation that maps the high-dimensional sensory information onto meaningful task states. This process requires not only distinguishing between relevant versus irrelevant input features, but also the incorporation of unobservable knowledge, such as prior contextual information, and knowledge about structural relationships between different objects. Area 1 is concerned with how the brain uses and learns abstract representations during value-based decision making, and asks how we can make good decisions in the face of highly complex and changing sensory observations.
The research we pursue in this area seeks to elucidate where, when, and how the human brain forms such a map of relations between events or items in a given environment in a quasi-geometric format that goes beyond simple stimulus-response learning and facilitates novel inferences. The particular approach we take lies at the intersection of (a) the idea of a cognitive map in the brain as envisaged by Tolman, (b) our knowledge of the neurobiology of value-based decision making, and (c) computational approaches to value and representation learning that have fueled much progress in AI.
This approach differentiates us from most other groups in the field. While most studies in this domain focus on the hippocampal formation, we broaden the concept of a cognitive map. We propose that multiple maps exist in both the hippocampus and prefrontal cortex, influencing each other, and focus on how learning these maps is driven by reinforcement learning signals (see, e.g., Garvert et al., 2023, described below). Contrary to the original animal work based on place cells, we also seek to establish that such maps are not restricted to spatial information (Wu et al., 2020). Based on our own previous work, we focus specifically on the role of the orbitofrontal cortex in building cognitive maps (Schuck et al., 2016), and study how the well-known value signals found in this same area integrate with cognitive map-like representations (Moneta et al., 2023 and see below). Finally, we take an integrated computational approach known as (deep) reinforcement learning, in which the development of internal representational spaces and value-signals both derive from learning operations in a connectionist architecture.
Key References
Project 1.1: Representation Learning of Task States
Research Scientists
Shany Grossman
Andrew Saxe (Gatsby Unit & Sainsbury Wellcome Centre, University College London, UK)
Christopher Summerfield (University of Oxford, UK)
Nicolas W. Schuck
In this study, we aim to investigate representation learning in the human brain through the lens of deep reinforcement learning networks. Using this computational framework, which combines scalar reward feedback signals with backpropagation and a connectionist architecture, we derive a number of testable predictions about learning-induced changes in human behavior and brain activity that we aim to test using fMRI.
To this end, we designed a partially observable decision-making task, in which a context (a client) determines the values of a pair of choice options (houses) that are composed of two relevant (i.e., reward-predicting) and one reward-irrelevant feature. Participants learn from feedback, and can only be successful at the task if they develop context-dependent and attentionally filtered house representations (see Figure 1a/b for the task description).
Results from a recurrent neural network (Figure 1c) trained to predict the values of each client–house combination in our task show that the learned internal representations of task states are primarily organized along two orthogonal axes—client type (i.e., context) and house type (Figure 1d). Interestingly, the network’s representational space primarily retains information about the input structure and does not merely collapse states based on their predicted reward, as might have been expected. Tracking the emergence of this representational space using a temporally resolved analysis, we find that the context—which explains the most variance in the hidden units’ responses (layer 1)—is the first to emerge during representation learning, followed by the specific combination of houses to choose from (Figure 1e), which differentiate also between relevant and irrelevant features. Values, however, are not a main driving force while learning task representations (although they are embedded more indirectly in the network activations, which can only be seen with a non-linear decoding approach). One interesting theoretical implication of a deep reinforcement learning account is that learning should show a particular covariation structure, meaning that feedback about one choice will influence future behavior not only in regards to this choice but also a number of related choices, as well as their internal representations. Our simulations show that this covariation structure indeed exists, and mimics the geometry of the network’s internal representations: Feedback about one state drove representational changes not only in this state but also in all other, non-feedbacked, states from the same context (Figure 1f). Ongoing work will test these predictions about state representation learning in behavioral and neural data (BOLD fMRI) from humans.
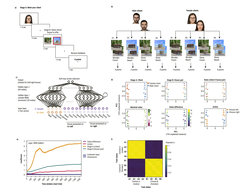
Figure 1. Representation learning of task states in a deep reinforcement learning model. (A) One single trial in the task. Participants are presented with an image of a client, followed by two optional houses they can offer the client. Upon selecting a house, participants received reward (how “pleased” the client was). (B) Value structure of the task: One relevant client dimension (female/male) and two relevant house dimensions (house type: wooden/stone; location: forest/beach) determined the received reward, while additional dimensions were reward-irrelevant (e.g., client with versus without glasses; house with pool versus without pool). (C) Neural network model trained on the task, trained with reward feedback (not supervised feedback) to predict the received reward, using stochastic gradient descent. Client and house information was fed into the network at two separate time steps, and information about the client was retained through recurrent connections in the network’s first hidden layer. (D) PCA of single trial representations in the first hidden layer (one representative simulation). The same plot is presented under different color codes, each marking a different trial division, see legend. (E) Using a time-resolved representational similarity analysis we tracked the impact of different factors on the hidden representation in the network throughout learning. (F) A pairwise matrix summarizing the correlations between representational changes of different states in the absence of any direct feedback to the measured states. A representational change in a state drives all states from the same context in the same direction, whereas states from the other context remain unaffected.
Project 1.2: Effects of Reward of Neural Representations of Spatial
Research Scientists
Nir Moneta
Charley Wu (University of Tübingen, Germany)
Christian F. Doeller (MPI for Human Cognitive and Brain Science, Leipzig, Germany)
Nicolas W. Schuck
Animals and humans maintain a cognitive map of the environment with unique spatial representations, including hippocampal place cells that encode specific locations and entorhinal grid cells that fire at regular intervals as the animal navigates. These and other cell types are generally seen as a coordinate system for spatial navigation, as well as abstract spaces (see Kaplan et al., 2017; Sharpe et al., 2018). Notably, cognitive maps are susceptible to reward locations, as shown for example by the findings that more place cells represent areas around reward locations, and that grid cells change in firing rate and location.
Project 1.2 aims to investigate how reward morphs cognitive spaces. In a first session, participants perform a perceptual discrimination task (Figure 2a). On each trial, a target tree is presented, followed by two reference trees, and participants need to judge which of the two reference trees is more similar to the target. Trees are characterized by the number of leaves and fruits they carry, such that each tree can be conceptualized as a particular point in a leaf/fruit space, and each comparison has an associated angle (target and references on a given trial always lie on the same line, see Figure 2b). Following this task, participants learn to associate a reward with a set of trees characterized by a specific number of leaves and fruits, akin to introducing a reward to a specific location in the cognitive map (Figure 2c). In the fourth and last session, participants repeat the initial discrimination task from the first session. fMRI is acquired during all sessions.
We hypothesized that at the moment of choice, when the reference trees appear, participants are traveling mentally between the three trees, akin to navigating in an abstract cognitive map. Initial fMRI pilot data reveals evidence for grid-like encoding during perceptual choices in the entorhinal cortex and distance-dependent encoding in the hippocampus. Behavioral analyses show that perceptual discrimination is enhanced for rewarded areas of the map but not for unrewarded ones. Our next steps are to test whether the behavioral change is due to a neural overrepresentation of rewarded areas. To this end, we plan to extract the subject-specific morphs of the cognitive map to test if hippocampal place encoding and entorhinal grid-like code adhere to the new, morphed map.
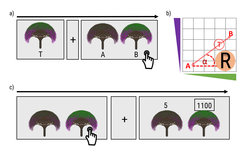
Figure 2. Task design. (a) The experiment consists of four sessions. Participants perform a perceptual discrimination task in the first and fourth sessions, where in each trial a similarity choice has to be made by indicating which of two reference trees is more similar to a target tree. (b) We hypothesize an underlying cognitive map where each tree can be mapped on by its number of leaves (x-axis) and number of fruits (y-axis). (c) Participants learned to associate a specific region in the perceptual space with reward in the second and third sessions. Participants were presented with two trees and had to make a free choice, after which a short fixation cross was presented, followed by the number of gold coins associated with the chosen tree. Note that in panel (b) the most rewarding area of the cognitive map is marked with R.
Project 1.3: Learning Slow, Learning Fast: Influence of Temporal Dynamics on Value-Based Function Approximation
Research Scientists
Noa Hedrich
Sam Hall-McMaster
Eric Schulz (MPI for Biological Cybernetics, Tübingen, Germany)
Nicolas W. Schuck
Learning what to attend to is a central part of representation learning. Interestingly, past machine learning research has suggested that meaningful information in the input data is often represented by features that change slowly over time, while fast variations may represent noise or less relevant information. This idea is encapsulated in the so-called slow feature analysis, which proposes that any a priori tendency to attend to slowly changing features of the environment can be an effective strategy for representation learning. We hypothesized that humans also make use of this principle by having a prior belief that slowly changing features are relevant for predicting reward.
Participants (n = 50) completed a learning task where they had to learn the rewards associated with visual stimuli characterized by two features, color or shape, that varied either slowly or quickly over time (Figure 3a–b). To ensure participants were aware of the feature differences, each block started with a passive observation phase in which sequences of changing stimuli were presented (Figure 3c). Crucially, participants then entered a decision-making phase, in which only one of the two features predicted reward, and participants had to decide on each trial whether to accept a default reward of 50 points, or receive the unknown reward associated with a shown stimulus (between 0 and 100 coins). Which feature was reward-predictive changed across blocks, such that in each block either the slowly changing or the quickly changing feature was task-relevant. Feature relevance had to be inferred by participants by observing the relationship between rewards and each feature. At the end of each block, knowledge of stimulus-associated rewards was probed by presenting pairs of stimuli not seen during learning and asking participants to choose the more valuable one, without feedback (Figure 3c).
As predicted, participants accumulated more reward, t(49) = 2.197, p1-sided = .016 (Figure 4a), and were more accurate in test trials, t(49) = 1.849, p1-sided = .035 (Figure 4b), when the relevant feature changed slowly instead of quickly. To understand the computational origins of this effect, we fitted participant choices with three reinforcement learning models that differed in how they adapted their learning rates to feature variability. One model used the same learning rate regardless of condition (1LR) and thus was indifferent to feature variability. Another model used different learning rates for blocks in which the relevant feature was slow versus fast (2LR), allowing it to adapt to the feature variability. The last model, in addition to adapting to variability, had separate learning rates for the relevant and irrelevant feature (4LR), which allowed it to learn only from the relevant feature, but also differentiate between relevant features that changed slowly versus quickly. Comparing AIC scores showed that the 2LR model performed best, with an average AIC of 475.7 (versus 479.3 and 476.3 for the 4LR and 1LR models, respectively). T-tests showed that AICs of the 2LR were significantly lower than the 4LR model, t(49) = -3.024, p = .012, Bonferroni corrected, but did not show a significant difference between the 1LR and 2LR models, t(49) = 0.422, p>.05 Bonferroni corrected (Figure 4c), reflecting large variability across participants (Figure 4d). The results indicate that humans learned better when the relevant feature was changing slowly, suggesting that a prior for slow features might improve human learning in multidimensional environments. Yet, there is significant individual variability in the extent of this effect. Data acquisition is completed and we are in the process of writing a manuscript. Data and code will be released upon publication.

Figure 3. Feature variance learning task. (a) Examples from the two feature spaces used in the experiment. Shapes were sourced from the Validated Circular Shape space and colors were defined as a slice in CIELAB color space. (b) Example sequence of bi-dimensional stimuli. Here shape is the slow feature (low trial-to-trial variability, similar shapes from trial to trial) and color is the fast feature (high trial-to-trial variability, dissimilar colors from trial to trial). (c) Schematic of one block of the task.
Image: panel (a) adapted from Li et al. (2020); all other panels by Noa Hedrich / MPI for Human Development
Original image licensed under https://osf.io/d9gyf/
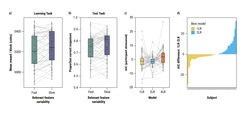
Project 1.4: Representation Learning Dynamics Underlying Aha-Moments
Research Scientists
Anika T. Löwe
LéoTouzo (Université Paris Cité, France)
Johannes Muhle-Karbe (Imperial College London, UK)
Andrew Saxe (Gatsby Unit & Sainsbury Wellcome Centre, University College London, UK)
Christopher Summerfield (University of Oxford, UK)
Nicolas W. Schuck
Project 1.4 is concerned with understanding moments of very fast learning, in which humans show large and sudden improvements in task performance that are accompanied by an aha-moment or insight. Insights have often been treated as a distinct phenomenon in the psychological and neuroscientific literature because they are preceded by a period of impasse, behavioral changes are unusually abrupt, and they occur selectively in only part of the population. These characteristics seem difficult to reconcile with neural network models that have become a prominent theory of human learning and can account for a wide range of learning phenomena. The gradient descent techniques at the heart of learning in these models seem particularly problematic, since they appear to imply that all learning is gradual.
We challenged this idea, and hypothesized that the aforementioned insight characteristics—suddenness, delay, and selectivity—do not necessitate a discrete learning algorithm but can emerge naturally within gradual learning systems. While present-day machines do not have meta-cognitive awareness, and therefore clearly cannot subjectively experience aha-moments, they might still show sudden behavioral changes even when trained gradually. To test our hypothesis, we compared human behavior in an insight task with neural networks trained using stochastic gradient descent. We focused on a simple feed-forward neural network architecture with regularized, multiplicative gates (Figure 5a). Gating can be seen as a mechanism that involves suppression of irrelevant input features, akin to top-down mechanisms found throughout the human brain. We stipulated that regularization of such gates might be the mechanism that leads to the impasse, i.e., the temporary blindness of some features that are key to a solution. Ninety-nine human participants and 99 regularized neural networks performed that same task, and networks were matched in performance to their human counterparts. The task required a binary choice about circular arrays of moving dots (scalar inputs for networks) and entailed a hidden regularity, which could be discovered through insight (Figure 5b). In line with previous work, we find a subset of human participants showed spontaneous insights during the task. Notably, a comparable subset of regularized neural networks also displayed spontaneous, jump-like learning that signified the sudden discovery of the hidden regularity (Figure 5c), i.e., networks exhibited all key characteristics of human insight-like behavior in the same task (suddenness, selectivity, delay). Gradual learning mechanisms hence seem to closely mimic human insight characteristics. Analyses of the neural networks’ weight-learning dynamics revealed that insight crucially depended on noise (Figure 5d), and was preceded by “silent knowledge” that is initially suppressed by (attentional) gating and regularization. Our results therefore shed light on the computational origins of insights and suggest that behavioral signatures of insight can naturally arise in gradual learning systems. More speculatively, in humans such nonlinear dynamics might be the starting point of conscious experiences associated with insight.
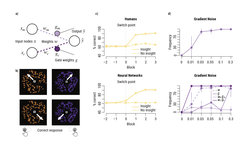