Humans and Machines
Director: Iyad Rahwan
Introductory Overview
Concept 2: Science Fiction Science
The Center for Humans & Machines (CHM) conducts interdisciplinary science to understand, anticipate, and shape major disruptions from digital media and artificial intelligence to the way we think, learn, work, play, cooperate, and govern.
Our goal is to understand how machines are shaping human society today and how they may continue to shape it in the future. We believe the challenges posed by the information revolution are no longer mere computer science problems.
In the following, we report on work carried out between 2019 and 2023. As the Center was founded in July 2019, this report also includes research conducted by CHM members formerly based at the Massachusetts Institute of Technology (MIT), as well as work done during the transition.
The Center explores various projects, organized into broad themes, which are diverse in terms of their scientific methodology and the research questions they explore. Each theme has an overarching research question, as shown in Figure 1.
A selection of completed and ongoing research projects in each theme will be outlined in detail below. First, however, we outline the overall scientific approach of CHM and its relationship to existing disciplines.
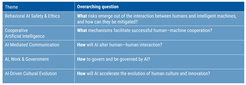
Figure 1. The current research themes of the Center span topics related to artificial intelligence.
Interdisciplinary Methods
The Center is decisively neither a traditional computer science department nor a traditional behavioral science department. Rather, it brings together scientists from diverse disciplines in order to shed light on the phenomena of interest (see Figure 2).
In particular, CHM employs (or will employ) scientists from three major groups of disciplines. First, computer scientists and data scientists provide the essential technical capability to produce the computational systems we are interested in studying (e.g., a reinforcement learning algorithm or a generative adversarial network) and to create technical measurement instruments (e.g., to collect social media posts from Twitter or to scrape online discussion forums and apply natural language processing techniques to them). However, our primary objective is not to contribute to the field of computer science directly in terms of new computational tools—although this does happen on occasion. This is evident in the fact that our primary publication venues are not computer science conferences and journals.

The second pillar of CHM are the social (behavioral) and cognitive sciences, which provide experimental methods and the theoretical foundation for understanding how humans interact with machines. We thus aim to hire highly qualified quantitative psychologists, political scientists, economists, biologists, and anthropologists.
Finally, the disciplines of physics and mathematical/statistical modeling provide an additional set of tools typically not available to the average computer scientist. This enables us to use tools from network science, dynamical systems, differential equations, and multilevel statistical modeling/Bayesian inference.
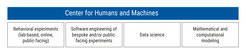
Figure 2. The research methodologies used at the Center build on diverse scientific disciplines.
Guiding Concepts
The Center is distinguished by the following three guiding concepts that help us identify and scope research questions. The Center has also been instrumental in the conceptual development and ongoing popularization of some of these concepts within the scientific community.
Concept 1: Machine Behavior
Understanding machine behavior, including human perception and reaction to such behavior, requires concepts and methodologies from across the behavioral sciences.

Figure 3. The foundational questions of machine behavior, inspired by Tinbergen’s four questions in biology.
Image: Springer Nature
Adapted from Rahwan et al. (2019)
The main focus of the Center is around the notion of machine behavior. We are interested in two broad aspects: (1) how intelligent machines behave, and the outcomes that emerge as machines interact with humans; and (2) how humans perceive the behavior of machines, and how this perception shapes their expectations and judgment of the machines’ actions and their own behavior. The contours of the emerging field of machine behavior were outlined in this article.
Despite fundamental differences between machines and biological organisms, we draw inspiration from Tinbergen’s four questions of biology in order to organize the different kinds of questions one might ask about machine behavior. Machines have mechanisms that produce behavior, undergo development that integrates environmental information into behavior, produce functional consequences that cause specific machines to become more or less common in specific environments, and embody evolutionary histories through which past environments and human decisions continue to influence machine behavior. These four levels of analysis are summarized in Figure 3.
Fundamental questions in machine behavior include the emergence of human–machine cooperation, potential social dilemmas and moral hazards that may arise from human–machine interaction, the potential role of machines as social catalysts, and the impact of AI on human culture.
Key References
Concept 2: Science Fiction Science
To anticipate the impact of future technologies on humans, we combine our imagination of possible futures with a scientific approach to studying behavior.
Science fiction allows us to imagine alternative future worlds shaped by scientific and technological breakthroughs, and to explore how they might change human behavior. But science fiction literature focuses on one narrative at a time. The fields of futures studies and design fiction anticipate future technological change more systematically, through codified design practices or qualitative and quantitative forecasting methods. It remains difficult, however, to anticipate the impact of these futures on human behavior.
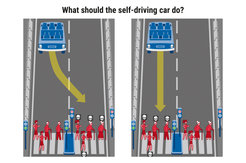
Figure 4. Screenshot of a random Moral Machine scenario.
Image: The Moral Machine Experiment
Science fiction science (SFS) attempts to simulate future worlds in order to test hypotheses about human behavior in those futures. It does so by combining the imaginative power of science fiction with the methods of behavioral science. For example, before fully autonomous vehicles (AVs) become a reality, one could use computer simulation of vehicle behavior and an understanding of human mobility to forecast how AVs might alter transportation behavior or greenhouse emissions. Similarly, one can systematically generate scenarios in which future AVs might face ethical dilemmas on the road, as in the Moral Machine experiment, to empirically study people’s judgment of such behaviors before such technology exists. This anticipatory approach allows us to test hypotheses about human reactions to future technologies, and to track those reactions over time.
In addition to simulating future scenarios, pursuing an SFS approach may require the invention of novel technologies in a proactive manner, or pushing existing technologies to new limits. For example, in the early days of social media, in order to understand whether this new medium was capable of achieving large-scale cooperation, an unprecedented feat of time-critical social mobilization had to be attempted, demonstrated, and then studied. As Nobel Prize winner and inventor of holography Dennis Gabor once wrote: “The future cannot be predicted, but futures can be invented.”
Key References
Concept 3: Superminds
The future will be determined by competition between superminds: groups of humans augmented by culture, institutions, communication technology, and artificial intelligence.
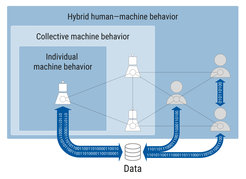
Figure 5. We are interested in studying the behavior of machines as they interact with human decisions at various scales.
The term culture describes information that affects the behavior of individuals, who acquire it from other members of their species through teaching, imitation, and other forms of social transmission. It is now increasingly recognized that humans have become the dominant species on Earth not through their individual intelligence, but through their ability to create and transmit adaptive cultural innovations (norms, institutions, technological know-how, etc.) through a process of cultural evolution (Henrich, 2016, The secret of our success: How culture is driving human evolution, domesticating our species, and making us smarter).
The Information Age is facilitating two monumental changes in human organization and culture. First, digital communication technologies, such as smartphones and social media, are massively expanding our ability to organize and coordinate at scale. Second, creative software is radically changing how we create, store, and share cultural information (Acerbi, 2019, Cultural evolution in the digital age). AI tools are facilitating new kinds of hybrid human–machine creative practices. Recommender systems are shaping how cultural information is disseminated. And generative AI software is even becoming an active “agent” in the culture generation process, via computer-generated artistic and technological inventions.
These two phenomena—cultural evolution and the information revolution—give primacy to emerging forms of human–machine collective intelligence. Consequently, it is likely that future human progress will be shaped by competition operating not among individual humans or groups of humans, but between hybrid human–machine collectives, or superminds (Malone, 2018, Superminds: The surprising power of people and computers thinking together). Hence, it is important to understand the drivers of collective intelligence (and stupidity) exhibited by superminds, as well as the dynamics of competition among superminds.