AI & the Future of Work
Sample Project: Link Prediction on Job Market (Ongoing)
How are machines impacting human jobs, organizations, and economies?
Robotics and artificial intelligence technologies are expected to fundamentally transform the nature of work, to potentially eliminate many jobs, and to create entirely new fields of work. While these questions are typically studied by labor economists, this theme complements the traditional approach using methods from data science, machine learning, complex systems, and network science to shed new light on these processes.
Sample Project: Longitudinal Complex Dynamics of Labor Markets Reveal Increasing Polarization (Ongoing)
How is the structure of job tasks changing over time?
In this project, we conduct a longitudinal analysis of the structure of labor markets in the US over seven decades of technological, economic, and policy change. We make use of network science, natural language processing, and machine learning to uncover structural changes in the labor market over time. We find a steady rate of both the disappearance of jobs and a shift in the required work tasks, despite much technological and economic change over this time period. Machine learning is used to classify jobs as being predominantly cognitive or physical, based on a written description of the workplace tasks. We also measure increasing polarization between these two classes of jobs, linked by the similarity of tasks over time, that could constrain workers wishing to move to different jobs.
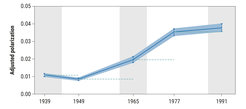
Figure 1. The polarization of the physical and cognitive tasks has been increasing over time in the US.
Image: MPI for Human Development
Adapted from Althobaiti, Alabdulkareem, Shen, Rahwan, Frank, Moro, & Rutherford (2022)
Original image licensed under CC BY 4.0
Key Reference
Sample Project: Link Prediction on Job Market (Ongoing)
This project uses link prediction techniques from network science in order to predict the emergence of future jobs.
Recently, research on the future of work has utilized network science to understand the structure of labor markets. This includes skills, jobs, and unemployment shocks with great success. However, despite this evidence that labor markets are strongly mediated by network structure, our understanding of how their structure evolves and how this informs public policy is limited.
In our studies, we build on these foundational network-based studies and apply advanced network science techniques to better understand the dynamics of these complex systems. Specifically, we implement link prediction methods from network science to predict the future occupation networks and labor market landscapes (Lü et al., 2015, Toward link predictability of complex networks). As shown in Figure 2, the job of tractor operator is connected to related jobs, e.g., truck driver and mining operator, due to the similarity of skills required; likewise, radiologists may share similar knowledge and skills—such as equipment operating, software, and knowledge of physics—with nuclear engineers and engineering technologists, thus they are connected in the occupation network. However, with the development of science, technologies like autonomous driving and image recognition software are widely accepted and adapted by a large number of occupations, and so tractor operator and radiologist could become increasingly similar jobs in the future: They may use similar software and instruments to make their respective tasks more efficient and automated.
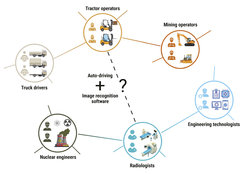
Figure 2. Jobs share similar skills. With the development of technology, is it possible that some currently different jobs, like tractor operator and radiologist, will become alike in the future?
Based on O*NET data, we build occupation networks and predict future links among jobs by link prediction methods such as the SPM method from network science. In Figure 2, the degree distributions in 2011 and 2020 and the predicted distributions by SPM in years 2030, 2040, 2050, 2060, and 2070 are presented. It can be observed that degree distributions are getting more uneven and degree variance keeps increasing, which may suggest occupations are becoming more heterogeneous and polarized (Alabdulkareem et al., 2018. Policies could potentially aim at building a more robust labor market with the future occupation contents shifting.
Meanwhile, we did not observe the preferential attachment (“the rich get richer”) phenomenon. Occupations with low initial degrees get more links in the future. This suggests that jobs that are relatively isolated and do not share many common skills with others will receive major opportunities to change their working styles and contents to build connections with other jobs. We could expect a more diverse labor market landscape. At the same time, by comparing the theoretical best algorithm performance, we could conclude that link prediction methods demonstrate that a fundamental limit to how the evolution of labor markets can be predicted exists (Sun et al., 2020, Revealing the predictability of intrinsic structure in complex networks).
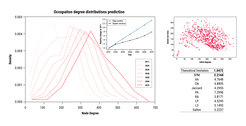
Figure 3. Left: degree distributions of occupation in 2011, 2020–2070; top right: new links versus initial degrees of all jobs; bottom right: theoretical limitation and theoretical best algorithm performance of link prediction methods.
Our research provides a foundational understanding of the labor market evolutions, which in turn dictates how and which jobs would be targeted when applying regulation that seeks to maximize social mobility, ameliorate the effects of skill-based technological automation, and incentivize structural economic change, e.g., green technologies. With new tools from network theories applied to occupation data, more detailed findings regarding the future of work could be expected to guide policy-makers.
Key Reference