Emmy Noether Group: Lifespan Neural Dynamics Group (Concluding Report)
Head: Douglas D. Garrett
Dopaminergic Bases of Age and Performance-Graded Differences in Signal Variability
Using Computational Models of Behavior and Brain to Understand Brain Signal Variability
The Lifespan Neural Dynamics Group (LNDG) has centered primarily on healthy cognitive and neural development across the lifespan. Specifically, our research investigates the computational and neurobiological bases for moment-to-moment fluctuations in brain activity (see Figure 1), with a key emphasis on cognitive aging (Garrett et al., 2013; Waschke et al., 2021). In contrast to earlier approaches that have historically assumed brain signal variability to be a form of detrimental “neural noise,” our research program indicates that moment-to-moment signal variability can instead facilitate neural communication, flexibility, and adaptability. Our work has been at the forefront of an emerging line of research demonstrating that older, poorer performing adults reliably exhibit less signal variability across moments than younger, better performing adults (within and across brain regions and task types), an agenda we increasingly pursue longitudinally. Based on this body of work, we have proposed that the field abandons traditional theoretical considerations of "neural noise" as a primary basis for aging-related cognitive deficits, and instead reconceptualizes age-related neurocognitive impairment as a generalized (and experimentally testable) process of increasing moment-to-moment rigidity and loss of dynamic range. Our research is inherently multidisciplinary, spanning computational and in vivo neuroscience, multi-modal neuroimaging, developmental science, cognition, psychiatry, statistics, and machine learning. LNDG research has been financially supported by a 6-year Emmy Noether grant from the German Research Foundation (DFG) to Douglas D. Garrett (2017–2023). Research highlights focusing on the reporting period are summarized below.
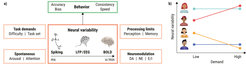
Adapted from Waschke et al. (2021)
Key Reference
Dopaminergic Bases of Age and Performance-Graded Differences in Signal Variability
Our research program has continued to expand along multiple principled axes. First, we are working to establish the dopaminergic bases of age and performance-graded differences in signal variability. Given that normal aging is associated with dopaminergic (DA) decline and poorer cognitive performance, we have examined whether commercially available pharmacological agents that upregulate systemic DA could restore deficient signal variability levels in older adults. Our first study revealed that older adults expressed lower signal variability on placebo yet matched or exceeded young adult variability levels in the presence of amphetamine, while improving in speeded performance upon first task exposure (Garrett et al., 2015). We recently found that another DA agonist (L-DOPA) also boosts blood-oxygen-level-dependent (BOLD) variability in older adults during reinforcement learning (Skowron & Garrett, in prep.), and that DA antagonism can reduce BOLD variability in younger adults (Garrett et al., in prep.). Further, we are core members of the COBRA Study, the world’s first large-scale, longitudinal dopamine positron emission tomography (PET) and fMRI study of older adults (N = 180, 64–68 years; Nyberg et al., 2016). In our most broad-scale COBRA effort to date, we are now attempting to demonstrate that the ability to upregulate circuit-specific neural variability under working memory load is a joint reflection of greater dopamine D2 capacity and more optimal working memory-based decision making. We also collaborate with several other international groups (e.g., at Duke University, at Brandeis University) on aging-oriented DA PET and pharmaco-fMRI studies, with a focus on the dopaminergic basis of brain signal variability in relation to decision making across the adult lifespan.
Using Computational Models of Behavior and Brain to Understand Brain Signal Variability
Second, we leverage multiple computational models of both behavior and brain for understanding the nature and functional utility of brain signal variability in younger and older adults. Beyond the use of reinforcement learning models of behavior in our work using L-DOPA noted above, we continue to utilize a series of other tailored behavioral models in our work. For example, our recent EEG work shows that the implementation of evidence accumulation biases during perceptual decision making (parameterized via Ratcliff’s drift diffusion model) are implemented within-person by modulating oscillatory “excitability” in response to reward contingencies (Kloosterman et al., 2019). We also find that those who maximize reward by elevating their evidence accumulation biases are those who maximally elevate EEG entropy levels immediately post-stimulus in prefrontal cortex, providing the first behaviorally relevant top-down signature of response bias in the human brain (Kloosterman et al., 2020). We are now following up this work in older adults to optimize age-related response biases via real-time feedback. Two PhD students in the group are also pursuing computational approaches to understanding (a) explore–exploit decision making using a series of AI-inspired behavioral algorithms, such as the upper confidence bound and Thompson sampling models (Dissertation Liliana Polanski); and (b) behavioral response variability during uncertainty/structured learning using a series of stochastic Bayesian updating models (Dissertation Alexander Skowron) in younger and older adults undergoing fMRI and eye tracking.
We further utilize various computational models of the visual cortex/image recognition in our work to examine key cognition- and aging-related questions. Inspired by the notion that the brain could conceivably limit resource allocation (narrowing neural dynamic range) when stimulus input is simpler/more reducible but upregulate dynamic range to encode more differentiated sources of sensory input, we have embarked on a series of studies attempting to link the complexity of visual input to neural dynamic range (variability). In our first study in this domain (Garrett et al., 2020), we used the visuo-cortically inspired HMAX multilayer computational model of object recognition to estimate the complexity of visual stimuli perceived by participants during fMRI. We showed that moment-to-moment BOLD variability in visual cortex indeed increased when confronted with more feature-rich visual input, an effect especially present in adults with better performance across 20 different cognitive tasks. We have recently expanded this line of work into the domain of human memory. Moving beyond fMRI, we are testing the link between stimulus complexity (using a combination of HMAX and broadly utilized deep neural nets for image classification, such as VGG-16) and neural variability in human multi-neuron spiking data. Using customized within-person latent models, we show that trial-wise coupling between image complexity and spiking entropy during visual encoding is boosted with increasing model layer depth, suggesting that hippocampal spiking entropy is likely more sensitive to aggregated, “abstract” visual features. Crucially, we then find that stronger coupling between visual complexity and spiking entropy at encoding yields better performance during memory recognition, across multiple behavioral metrics. We are also now targeting the neurochemical mechanisms that may drive such effects. Ongoing work reveals that those older adults best able to align brain signal variability to stimulus complexity also express higher visuo-cortical gamma-aminobutyric acid (GABA) levels, an inhibitory neurotransmitter presumed key for the differentiation of visual features. We have also shown that GABA agonism can upregulate signal variability in older adults with limited baseline GABA (e.g., Lalwani et al., 2021). We are thus beginning to establish GABA as a key neurochemical target when probing how humans utilize neural variability to adapt to the complexity of the visual world.
Key References
The Role of the Thalamus
Third, we are establishing the thalamus as a fundamental structure for understanding the interaction between local (regional) signal variability, large-scale networks, and behavior across the lifespan. Inspired by animal work demonstrating that local temporal variability may primarily reflect synaptic input rather than locally generated “noise,” our work provided first evidence showing that individuals with higher local temporal variability indeed had a more integrated (lower-dimensional) network fingerprint, and that thalamic variability was the strongest determinant of how the whole brain integrates from moment-to-moment (Garrett et al., 2018). Further, we continue to find that older adults who can increase functional integration within the striato-thalamic system under working memory load are better able to upregulate BOLD variability across the entire brain. Our longitudinal work in the NCMCA study suggests that those adults best able to maintain thalamic dynamics over a 2.5-year period are also best able to maintain performance in all major cognitive domains (see Figure 2; Garrett et al., 2021). Finally, in a landmark study for my group combining EEG, fMRI, eye tracking, and computational modeling of behavior (see Figure 3; Kosciessa et al., 2021), we found that when younger participants were more uncertain about what to process in their environment, cortical EEG signals shifted from a rhythmic to a more arrhythmic (“noisy”) mode, a phenomenon strongly rooted in the within-person regulation activity in specific thalamic nuclei (as measured by fMRI). Our results argue that the thalamus may support neural dynamics in general and could optimize brain states according to environmental demands, allowing adults to make better decisions. We are now preparing a manuscript showing that older adults with more young-like regulation of cortico-thalamic dynamics under uncertainty also maintain young-like behavioral performance. Broadly, our work supports a key role for the thalamus in how the brain fluctuates and communicates overall, providing a springboard for future tests of the thalamus (rather than cortex) as a fundamental determinant of aging- and cognition-related differences in brain dynamics. We take this stance formally in a recent review on human thalamic neuroimaging (Shine et al., 2023).
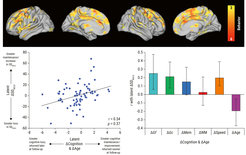
Image: MPI for Human Development
Adapted from Garrett et al. (2021)
Original image licensed under CC BY 4.0
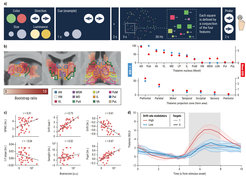
Image: MPI for Human Development
Adapted from Kosciessa et al. (2021)
Original image licensed under CC BY 4.0
Key References
Translational Approaches
Fourth, the LNDG’s research program is increasingly translational in several complementary ways. Beyond the translational potential of our work on the neurochemical bases of aging-related loss of brain dynamics, we are currently pursuing whether it is possible to “inject noise” into older brains to improve cognitive function. We have now initiated a series of studies that utilize real-time, closed-loop transcranial random stimulation (tRNS)-EEG solutions to determine adaptive, individually tailored “noise regimes” (e.g., specific noise colors and magnitudes) that improve visual perception in older adults. We also model such phenomena via a series of modified dynamical attractor models of bistable perception. One ultimate goal is to develop real-world, mobile/wearable technological solutions that allow invocation of tailored stimulation-based noise regimes across the lifespan. In another example of the translational capacity of our work, we use latent-level machine learning techniques to study whether pre-treatment brain signal variability can act as a reliable predictor of longitudinal cognitive behavioral therapy and drug-based treatment outcomes in samples of younger and older social anxiety patients in Stockholm. Our most recent results suggest that baseline BOLD signal variability models provide a 20–30% improvement in treatment outcome prediction compared to gold standard baseline measures of social anxiety disorder symptoms (Månsson et al., 2022). Our follow-up work is now testing whether baseline signal variability can predict which specific treatment patients should receive (e.g., cognitive behavioral therapy versus medication with selective serotonin reuptake inhibitors, SSRI).
Key Reference
Open Science
Finally, we continue to be strong supporters of open methods/science. We make complete and carefully documented data sets publicly available whenever possible (see Kosciessa et al., 2021). We also remain committed to keeping our customized algorithms/methods freely available (e.g., the Variability Toolbox (VarTbx) for SPM). We are active and open developers of signal processing techniques, such as oscillation detection (Kosciessa, Grandy, et al., 2020), variance-independent implementations of entropy estimation (Kloosterman et al., 2020; Kosciessa, Kloosterman, et al., 2020), and multivariate/multi-modal methods. All code and analysis pipelines/tools we utilize within the Lifespan Neural Dynamics Group are available on GitHub and applicable to a wide range of neuroimaging data collected at the MPI for Human Development and beyond.