Adaptive Memory and Decision Making Group
Head: Bernhard Spitzer
The Dynamic Representational Nature of Working Memory
“Optimal Irrationality” in Human Decision Making and Learning
Our current understanding of higher cognition faces a conundrum. On the one hand, humans and other animals show remarkably adaptive behaviors, successfully navigating our ever-complex world and accomplishing tremendous feats of intelligence. On the other hand, biological brains are inherently capacity-limited: The brain can process only a little information in a given time, and any neural processing is fundamentally noisy. The newly established Adaptive Memory and Decision Making Group (AMD Group; principal investigator: Bernhard Spitzer) uses computational and neuroscientific approaches to shed new light on this apparent contradiction in core cognitive functions such as decision making, learning, and working memory.
The research group has evolved from the former research area Neurocognitive Foundations of Adaptive Rationality at the Center for Adaptive Rationality (2018–2021). In 2022, the group was established as an independent unit led by Bernhard Spitzer, funded by an ERC Consolidator Grant (2022–2026), a German Research Foundation (DFG) Heisenberg Award (2022–2027), and additional funds from the DFG (2021–2024). Following delays in the wake of COVID-19, the first PhD students and postdocs joined the group in May 2022. Since September 2022, the group also hosts a PhD student of the International Max Planck Research School LIFE, Dilara Zorbek.
The Dynamic Representational Nature of Working Memory
One of the main limitations of human cognition seems to be the strictly limited storage capacity of working memory. Nevertheless, working memory excels in keeping ready the momentary contents of all of our thinking. How the brain accomplishes this feat, beyond the temporary maintenance of just experienced information, is hardly understood. An emerging view suggests that working memory storage is topographically distributed in the brain according to the information’s endogenous level of abstraction. However, very little is known about how such distributed storage is orchestrated spatiotemporally, that is, how representations at different levels of abstraction are structured dynamically in time to suit current demands. In its ERC-funded research program, the AMD Group uses tailored experimental designs and newly developed multivariate analysis techniques to explore how working memory dynamically changes its contents’ level of abstraction depending on momentary task requirements.
To quantify levels of abstraction in the brain, the group examines the extent to which neural activity patterns generalize across different stimulus spaces while task-relevant stimulus information is maintained in working memory. Throughout the research program, functional imaging (fMRI), electro- and magnetoencephalography (EEG, MEG), and invasive neural recordings will be used jointly to track levels of abstraction spatiotemporally, with millisecond precision. At the time of this report, the group is in the process of collecting EEG and fMRI data to test one of the more provocative hypotheses of the research program: that it may not be of foremost importance how much information working memory can store, and at which precision. More critical might be working memory’s enormous flexibility to provide information at just the right time, and in just the right format, that is, at an appropriate level of abstraction.

Adapted from Linde-Domingo & Spitzer (2022)
Original image licensed under CC BY-NC-ND 4.0
While the work on the ERC-funded project has only just started, promising new insights have already been gained during the piloting of the first set of studies. In piloting, the group routinely uses high-precision eye tracking to manage the risk that eye artefacts may pose for the interpretability of subsequent neural recordings (e.g., EEG). However, eye-tracking data also provide an ideal testbed for the group’s methodological framework: The multivariate analysis techniques developed for the program can be directly applied to two-dimensional eye-tracking data, just as they will later be applied to high-dimensional (and thus much more complex) brain signal patterns. Preliminary results (Linde-Domingo & Spitzer, 2022) have already provided first support for the research program’s key hypotheses: specifically, that the representational format of task-relevant information in working memory may change (a) rapidly and (b) adaptively, depending on momentary processing demands. These preliminary findings are in line with another early study from the group, which examined behavioral dynamics (response times) to show that the format of working memory storage may change abruptly when other stimuli need to be processed at the same time (Kerrén, Linde-Domingo, Spitzer, 2022).
Over the coming years, the group expects to extend these preliminary insights to the level of brain processing. Specifically, the AMD Group seeks to shed new light on how neural representations of working memory information are modulated by attention, inattention, and distraction, and how multiple contents are stored simultaneously. The goal of this work is to shape a new theory of the neurocognitive capacity limit in working memory. Further, novel hypotheses about how working memory interfaces with long-term memory and how it develops over the lifespan will be tested. Finally, the group’s human neuroimaging approaches will be combined with direct electrophysiological brain recordings in non-human primates during the same tasks, to disclose the fine-grained neural mechanisms of dynamic abstraction down to the single-cell level. It is anticipated that the program will provide new insights into the dynamic and multilayered nature of working memory, beyond the number and precision of items it can hold.
Key References
“Optimal Irrationality” in Human Decision Making and Learning
Given the limited capacity of working memory, how can humans still (a) learn efficiently and (b) make adaptive decisions? How does our cognitive machinery counter the fact that it operates only on a severely limited amount—and reliability—of information from the outside world? Decades of descriptive analysis have suggested that human decisions are irrational, and that human decision-makers over- or underweight objective evidence in idiosyncratic ways. But what if such “distorted” behaviors are not just idiosyncratic quirks, but have evolved as effective adaptions of an efficient low-energy system to its own computational limitations?
In a previous line of work, the group’s principal investigator has demonstrated that common psychometric distortions, such as the over- or underweighting of extreme values in numerical judgments, can paradoxically improve the performance of a noisy (i.e., capacity-limited) observer. This framework of “optimal irrationality” has already been successful in accounting for over/underweighting of numerical evidence in sequential decisions (Spitzer et al., 2017; Clarmann von Clarenau et al., 2022), for the distortions of value- and probability information in Prospect Theory (Juechems et al., 2020), and for adaptive learning strategies in solving transitive inferences (Ciranka, Linde-Domingo et al., & Spitzer, 2022). In all these domains, it was found that seemingly irrational human behaviors afford objective performance benefits over policies that seem normatively ”rational" but are poorly adapted to the computational and metabolic limitations of biological brains. Throughout its experimental work of the group, the AMD Group seeks to scrutinize these fascinating ideas and models in new domains, from basic working memory operations (cf. above) to memory-based decisions and risk-taking behaviors.
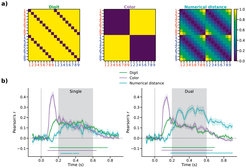
Adapted from Appelhoff, Hertwig, & Spitzer (2022)
Original image licensed under CC BY 4.0
At the time of this report, the group is particularly focused on extending its recent new insights into transitive inference learning. Transitive inference refers to the capacity that after learning pairwise relations, such as A>B and B>C, humans can infer that A>C. Members of the research group have shown previously that such transitive inference is facilitated by a learning “asymmetry,” where participants either attend only to the “winner” (e.g., A) or to the “loser” (e.g., B) of a pairwise comparison (Ciranka, Linde-Domingo et al. & Spitzer, 2022). Interestingly, asymmetric learning is also working memory-efficient, because it allows the discarding of parts of the experienced information. In ongoing and future work funded by the DFG, the AMD Group continues to investigate the ramifications of such asymmetries from the perspective of model-free and model-based learning, including on the level of neural processing. In addition to advancing previous psychological and neuroscientific theories, it is anticipated that the results from this work will be of strong relevance for the fields of machine learning and artificial intelligence, where the problems of transfer and flexibility—at high speed and low energy consumption—remain foremost challenges.
Key References