The Collective Mind
Reducing Antibiotic Overprescription by Harnessing the Wisdrom of Crowds
Strategies for Integrating Disparate Social Information
Cognitive Mechanisms Underlying Information Cascades
A Computational Theory of Conflicts in Groups
Joining a Group Diverts Regret and Responsibility Away From the Individual
Adaptive Social Networks Promote Collective Intelligence
Modeling Echo Chambers and Polarization Dynamics in Social Networks
Our decisions are typically not Robinson Crusoe’s decisions, and even he operated in a social world after Friday’s arrival. Social worlds provide us with the chance to improve our judgments and make better decisions. Unfortunately, living in a social world also carries the risk of being influenced by the “madness of crowds,” which may, for instance, foster the spread and amplification of misinformation. In this section, we report on our ongoing work aimed at better understanding how we can harness collective intelligence to provide a social good, for instance, better healthcare outcomes, and the cognitive strategies underlying the integration of social information. Then we move to the group level, providing a computational theory of what a group representation is in the context of conflict, and we investigate the reasons for joining a group in the first place. Finally, we present our work at the level of social networks, showing how the dynamics across adaptive networks can increase the quality of decisions but also lead to radicalization.
Reducing Antibiotic Overprescription by Harnessing the Wisdom of Crowds
The overprescription of drugs is a major global problem and costly in terms of financial and health consequences. The problem is especially pronounced with antibiotic prescriptions. Their overprescription contributes to rising levels of antibiotic resistance and patient mortality. To gain insight into this thorny issue, we (Krockow et al., 2020) investigated whether a “wisdom of crowds” perspective could promote an approach to prescribing antibiotics that is more compliant with medical guidelines (i.e., typically shorter durations). We used international survey data from 787 expert antibiotic prescribers from around the world. Across various clinical scenarios, they independently provided recommendations for treatment duration. We tested several aggregation algorithms to evaluate how pooling the independent decisions of the experts could improve antibiotic guideline compliance. We found that pooling the treatment recommendations improved guideline compliance across almost all clinical scenarios. This effect emerged in groups of just three expert prescribers and grew stronger in larger group sizes.
Most previous interventions to reduce unnecessary antibiotic prescription relied on either restrictive techniques to deter overprescribing of antibiotics or persuasive approaches to encourage a more deliberate decision process by providing more information about the therapeutic decision process and the societal risk of antimicrobial resistance. The effectiveness of these approaches, however, varied. Our results suggest that pooling independent judgments can play a role in global antibiotic stewardship specifically, and in better medical decisions in general.
Key Reference
Strategies for Integrating Disparate Social Information
The cosmos of social information around us typically consists of multiple opinions, which differ in the degree to which they conflict with each other and with our own personal information. How do we integrate such different pieces of social information? To test this, we (Molleman et al., 2020) investigated what strategies people use when weighting diverse social sources. In our experiment, participants were briefly shown an image and asked to estimate the number of animals in it. Afterward, they were presented with three different estimates from other participants, which also differed from the participant's own estimate. This simulated a wide distribution of social information. Finally, participants could revise their initial estimate.
By applying a cognitive model of the integration of social information, we discovered two main factors that influence how people integrate different opinions. People were more likely to incorporate social estimates that were (a) close to their own personal estimate or (b) close to the other social estimates. If participants observed even just one social estimate close to their own, they tended to ignore the other estimates. Our findings can help us better understand how people form an opinion in light of conflicting social information and offer insights into the causes of opinion polarization.
Key Reference
Cognitive Mechanisms Underlying Information Cascades
The timing of decisions can have a major influence on how collective dynamics play out, from when pedestrians cross at a red light, to financial filter bubbles, to the spread of news online. To gain a better understanding of the cognitive mechanisms involved in the spread of information, we (Tump et al., 2020) posed a sequential decision-making task to groups of people. Participants first viewed a noisy stimulus and then indicated their own judgment and confidence. Afterward, they had the opportunity to reconsider their judgment while viewing the choices of their group members in real time. Participants could either make a decision quickly without social information or wait and observe the choices of others. Our findings show that the groups self-organized themselves, with confident and more accurate individuals deciding earlier, thereby providing high-quality information to their slower-moving peers.
We applied an evidence accumulation analysis to shed light on the cognitive mechanisms. We found (Figure 1) that in the social phase, more confident participants began with a more extreme starting point. The social influence was captured by a change in the drift rate. A participant’s willingness to wait for the choices of others was captured by the decision threshold. Our findings demonstrate how essential aspects of the cognitive underpinnings of social interactions influence information spread in social systems, paving the way for a more in-depth understanding of social systems.

Figure 1. Illustration of the evidence-accumulation process during the social phase with five individuals. The start point of each individual is influenced by their personal judgment and associated confidence. Highly confident individuals start closer to either of the thresholds (red lines) and decide earlier, providing social information for undecided (less confident) individuals by biasing their evidence accumulation (as indicated by the arrows pointing upward).
Key Reference
A Computational Theory of Conflicts in Groups
Understanding the psychology of intergroup conflict is one of social science’s most pressing goals. Although the phenomenon of “groups” has been studied for over a century, with several recent computational modeling and game theoretic innovations, we still lack literal (as opposed to metaphorical) descriptions of what the mind is representing when it represents a social “group.”
We (Pietraszewski, 2022) surveyed the history of scientific approaches to the study of groups. We argue that existing approaches fail to coalesce into a mechanistic description of how the mind represents and reasons about groups if we fail to generate explicit theories of what these representations may be (this is the “computational theory”). We present a viable candidate theory: that the purpose of a group representation at a mechanistic level (i.e., its “functional role”, in philosophical terms) is to assign individual agents to a specific set of roles within a small number of triadic interaction types (Figure 2). These roles-within-triadic-interaction types can represent any conflict dynamics involving three or more agents. This resolves a long-standing problem of reconciling the infinite generativity of social dynamics with a finite set of cognitive representations. We discussed the empirical predictions of this theory and the new enterprises that need to commence within the study of groups if we are to have adequate, mechanistic theories.
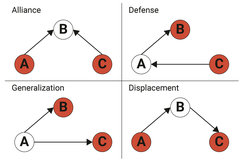
Figure: Cambridge University Press
Adapted from Pietraszewski (2022)
Key Reference
Joining a Group Diverts Regret and Responsibility Away From the Individual
Much of the research on collective intelligence focuses on when and how groups make better (or worse) decisions than individuals. An equally important but less researched topic is why individuals join groups in the first place. Why would we make a decision with a group rather than on our own? We (El Zein et al., 2020) examined this question through the lens of regret and responsibility, testing whether shared responsibility is a motivation to join a group, as it may help minimize negative emotions such as regret.
In our experiment, participants had to repeatedly choose between two lotteries that could win or lose them money. To induce regret, they saw the outcomes of the lottery they chose and the one they did not. Seeing the counterfactual outcome exposed them to what could have been, leading to the feeling of regret if it was better than what they had received. At each round, participants could play the lottery alone or in a group of five, following a majority vote. We found that the experience of loss and regret motivated people to join groups. The bigger the loss, the more likely they were to switch from playing alone to with others. When playing in a group, the outcomes did not matter as much for subsequent decisions. Negative outcomes experienced alone led to worse choices than positive outcomes, but this effect was absent when playing in a group. This suggests that the collective can reduce the influence of negative emotions and help individuals reevaluate past choices.
Key Reference
Adaptive Social Networks Promote Collective Intelligence
Our friends, relatives, and the people we follow on social media have a strong impact on what information we receive and how we form beliefs. Our social environment, however, is not static: It changes continuously as new ties are created and existing ones fade. But is this plasticity of social networks beneficial or detrimental to collective intelligence? Whereas most studies considered static social environments, we (Almaatouq et al., 2020) studied whether social networks can adapt to leverage the individual abilities and promote collective intelligence. For this, we conducted an experiment where groups of participants were placed in a communication network in which every group member had three neighbors. Participants undertook repeated estimation tasks with the possibility to see the answers of their neighbors. At the end of each round, the correct answer was shown and participants were able to replace some of their neighbors with other group members. That is, the structure of the network was malleable.
We found that social networks can quickly adapt and form collective intelligence. Across trials, the networks were more likely to centralize around the best-performing individuals. This resulted in better collective judgments than those of static networks and even surpassed the best-performing individual's performance. This demonstrates the “wisdom of the network,” with social-network plasticity as a key factor for refining collective judgments.
Key Reference
Modeling Echo Chambers and Polarization Dynamics in Social Networks
There is an ongoing debate about the nature of “echo chambers” on social media. A core component of echo chambers is the formation of homogeneous social networks of people with the same opinions. An unresolved question is whether people with similar opinions select into such groups or whether they collectively homogenize their opinions after joining such groups. We (Baumann et al., 2020) formalized a mechanism for the coevolution of these two processes, where opinions adapt to social contacts and social contacts adapt to opinions. In an agent-based simulation, microscopic rules specified that agents had a slight preference for like-minded people for their social interactions and that they adapted their opinions according to their social contacts. This enabled us to reproduce the macroscopic opinion distributions from real Twitter data on controversial topics (Figure 3).
Our model links the degree to which topics are controversial to the stability of these macroscopic states: When a topic allows for little “middle ground,” polarized opinion distributions and highly homogeneous network structures develop simultaneously. Our results shed light on a possible mechanism behind the emergence of echo chambers and polarization on social media regarding controversial topics: Namely, both the social network and the opinions of the agents are constantly adapting and thus spiral each other into the extreme states of opinion and segregated networks that can also be empirically observed (see Figure 3).

Figure 3. Contour maps show the average opinion of the closest neighbors to a user (⟨x⟩NN) versus the user's opinion (x) for simulations of the proposed model (Panel a) and three different data sets from real discussions on Twitter (Panels b–d). The colors indicate the density of users: The lighter the color, the more users there are. Additionally, the marginal distribution of opinions P(x) and the average opinion of the nearest neighbors PNN(x) are plotted on the x and y axis, respectively.
Figure: American Physical Society
Adapted from Baumann et al. (2020)
Key Reference