Behavioral AI Safety & Ethics
Sample Project: AI Advisor (Ongoing)
Sample Project: Delegation to AI (Ongoing)
Sample Project: Fear of AI Across Cultures (Ongoing)
What risks emerge out of the interaction between humans and intelligent machines, and how can they be mitigated?
Today, we increasingly interact with machines powered by artificial intelligence. AI is implemented even in the toys kids play with. AI powers home assistants like Amazon’s Alexa, which increasingly manages the lives of its over 100 million users. AI also engages in a growing array of tasks on behalf of humans, ranging from setting prices in online markets to interrogating suspects.
Key questions that the research area Behavioral AI Safety & Ethics tackles are: Could machines be bad apples and corrupt human behavior? How should we design AI systems to avoid ethical and safety risks? And how do people around the world perceive these risks?
Three projects that tackle the question of how AI systems shape human ethical behavior are AI Advisor, Delegation to AI, and Fear of AI Across Cultures.
Sample Project: AI Advisor (Ongoing)
While people disregard AI advice that promotes honesty, they willingly follow dishonesty-promoting advice, even when they know it comes from an AI.
The AI Advisor project examines whether and when people follow advice from AI systems that encourages them to break ethical rules. The motivation for this project comes from the observation that people receive ever more recommendations and advice from algorithmic systems. Research on recommender systems has a long-standing tradition.
The majority of research examines recommender systems such as YouTube or Spotify algorithms that suggest which videos to watch or songs to listen to. But algorithmic advice increasingly appears in the form of text seemingly written by humans. This human-like appearance of AI advice is made possible by recent advances in the AI domain of natural language processing (NLP), dealing with building algorithms to read, understand, and interpret human language. These NLP models have skyrocketed in size and scope and are increasingly implemented in systems that advise people what to do. Just to name a few examples: In the workplace, software applications like Gong.io analyze employees’ recorded sales calls and advise them in real time on increasing their sales. In private life, advice from AI systems grows, too. According to Amazon’s chief scientist Rohit Prasad, Alexa’s role “keeps growing from more of an assistant to an advisor.” In his view, people will soon not just request Alexa to play some jazz music but will also consult Alexa about whether they should break up with their partners or cheat on their tax claims. First evidence suggests that advice from these systems can go awry. Consider the recent outcry about the situation where a 10-year-old girl asked Alexa for a fun challenge, to which Alexa replied, “Stick a penny into a power socket!” Moreover, suppose algorithmic advisors like gong.io are programmed to maximize profits; in this case, such algorithms could recommend employees break ethical rules, for example, by lying to a customer about a product. This is not a hypothetical concern. Already in 2017, a team of Facebook researchers showed that NLP algorithms autonomously learned that deception is a viable strategy for negotiations. Yet, do people actually follow such AI advice?
In this project, we explore this question with behavioral experiments in which participants receive human-written and algorithmically generated advice before deciding, when completing an incentivized task, whether or not to break the ethical norm of honesty to gain financial profit (see the design in Figure 1). We further test whether the commonly proposed policy of making the existence of algorithms transparent (i.e., informing people that the advice comes from an algorithm and not a human) reduces people’s willingness to follow it.
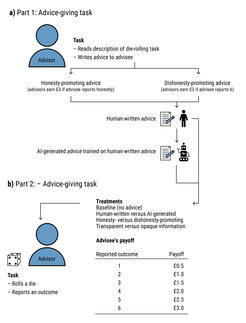
The results suggest that algorithmic advisors can act as influencers when people face ethical dilemmas, increasing unethical behavior to the same extent as human advisors do (see results in Figure 2). The commonly proposed policy of algorithmic transparency was not sufficient to reduce this effect.
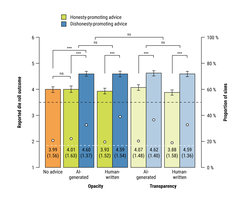
Sample Project: Delegation to AI (Ongoing)
Algorithmic delegation lowers people’s moral preferences, especially when the algorithms are programmed via supervised learning and goal-based programming.
The Delegation to AI project looks at another way algorithmic systems might influence humans’ ethical behavior: through delegation. The motivation for this project stems from the increasing practice of delegating tasks to algorithms. One commonly experienced example is the “smart pricing” option on Airbnb that enables people to let an algorithm set the rent price for their apartment. There is concern that these algorithms might break legal or ethical rules, even without humans being aware of it. For example, recent evidence suggests that pricing algorithms can collude autonomously. That means that algorithms, even when they are not programmed to do so, coordinate to set prices that are damaging to consumers. This has been documented in the laboratory and the field. Algorithmic collusion is receiving much policy interest. However, the human side of the equation is underexplored.
Theoretically, algorithms can act as enablers, allowing people to delegate unethical behavior to algorithms. Thus, new ethical risks might arise from algorithmic delegation. In this project, we test how people’s moral preferences shift when they can delegate ethical behavior to algorithms, and how the way in which the algorithms are programmed influences people’s moral preferences. The results reveal that people lower their moral preferences when they delegate to algorithms (versus humans). In two follow-up studies, we take a closer look at the way the algorithms are programmed. We compare three of the most common ways to program algorithms. One group of participants specified the if-then rules for the algorithm to follow. We call this rule-based programming. Another group of participants chose a data set of incomplete reporting profiles to train the algorithmic delegate. This mimics supervised learning. A third group specified the algorithm's goal, whether it should maximize honesty or profits in the die-rolling task (see design overview in Figure 3).
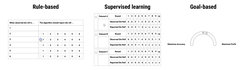
Figure 3. Overview of the different treatments that participants were assigned to. Left: In the rule-based programming treatment, participants specify which die roll to report for each observed die roll. Center: In the supervised learning programming treatment, participants choose a data set on which to train the algorithm. Right: In the goal-based programming treatment, participants specify the goal by setting the algorithm within a range from maximum accuracy to maximum profit.
The results confirm that algorithmic delegation lowers people’s moral preferences but also reveal that the way in which the algorithm is programmed matters. We find that around 5% of people who self-report the die-roll outcomes lie (see results in Figure 4). In the rule-based treatment, around one quarter of the participants programmed the algorithm to cheat; in the supervised learning treatment, almost half of the participants programmed the algorithm to cheat; and in the goal-based treatment, cheating levels rose to 87.6%. Psychologically, this form of training provides some plausible deniability. People can make themselves and others believe that they did not know the algorithm would break ethical rules.
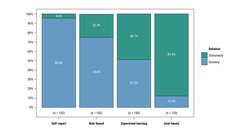
Figure 4. Results of Study 2, showing stark differences of dishonesty across treatments. The proportion of participants who acted honestly across all ten rounds is shown in blue and those who engaged in dishonesty shown in green.
These projects on behavioral AI safety provide insights into the ethical behavior of humans in interaction with and through real algorithms.
Key References
Köbis, N., Starke, C. Edward-Gill, J. The corruption risks of Artificial Intelligence. Transparency International Policy Paper. https://knowledgehub.transparency.org/assets/uploads/kproducts/The-Corruption-Risks-of-Artificial-Intelligence.pdf
Werner, T. (2022). Algorithmic and human collusion. Available at SSRN 3960738.
Sample Project: Fear of AI Across Cultures (Ongoing)
Fear of AI varies across countries but can be universally explained by a psychological model specifying local people’s expectations and their perception of AI minds.
There are good reasons to be worried about the deployment of AI in new occupational roles: As research in AI ethics repeatedly shows us, whenever AI is deployed in a new occupation, adverse effects can follow (Bigman & Gray, 2018, People are averse to machines making moral decisions). An important task is finding a way to minimize adverse effects, maximize positive effects, and reach a state where the balance of effects is ethically acceptable. Finding this balance is not enough, though, since the technology has to be accepted and adopted by the public. As a result, another important task is to measure, understand, and address the fears and psychological barriers experienced by the public. While important progress can be achieved gradually by studying the fear that participants from a single country have about a single application of AI, it would be an improvement to formulate and test a psychological model that would predict the fear that participants from different countries would have about the introduction of AI in different occupations. Though numerous studies suggest that people’s interaction with intelligent machines is comparable to social interaction with other people, regarding their psychological dimension, most of these studies were conducted in Western countries (Gray et al., 2007, Dimensions of mind perception; Waytz et al., 2010, Causes and consequences of mind perception). Here, we formulate and test one psychological model, which may have the potential to predict fear of AI across application domains and cultural contexts.
We recruited N = 10,000 participants from 20 countries spanning different continents, and asked people questions about (a) psychological requirements, (b) AI potential, and (c) fears about AI, regarding six human occupations that may be taken over by AI and have already triggered public concerns (doctor, judge, manager, care worker, religious worker, journalist). We expect that the mismatch between the psychological requirements of an occupation and AI’s perceived potential to fulfill these requirements will predict fear about deploying AI in the respective occupation.
We observe the expected relation in 17 out of 20 countries studied, as shown in Figure 5. In those 17 countries, there is a clear correlation between the fear of seeing AI deployed in a given occupation and the perceived potential of AI to display the psychological traits required for this occupation. However, China, Japan, and Turkey do not show this expected pattern, since these three countries also show the least fear about AI in our study. Beyond the descriptive value of our data set and the theoretical value of our model, our results can inform the efforts of policy-makers to communicate about AI with their citizens in a scientifically sound yet culturally sensitive way. If, for example, citizens in a given country are worried about AI doctors because they think AI does not have the high level of sincerity they expect from human doctors, then policy-makers may address this concern by implementing AI in a way that supports rather than replaces human doctors, or by increasing the transparency required of medical algorithms.
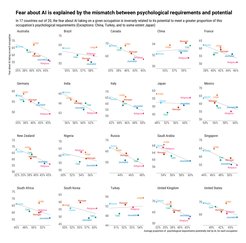
Figure 5. Fears of AI in the 20 countries, as a function of the proportion of AI’s matched psychological requirements across the six occupations.