AI-Mediated Communication
Sample Project: Lie Detection (Ongoing)
Sample Project: Blurry Face (Ongoing)
Sample Project: Minimal Turing Test (Ongoing)
How will AI alter human–human interaction?
Information and telecommunication technology has increasingly mediated human communication over the 20th century, from the telegram to the telephone, and from the cell phone to the Internet. The 21st century marks a qualitative shift in this technological mediation, namely, that the contents of communication itself are altered algorithmically, giving a new meaning to Marshall McLuhan’s famous phrase “The medium is the message.”
AI-mediated communication is already pervasive. The images we share online are altered through filters that enhance our appearance. Spellcheckers and AI-powered grammar checkers make sure our sentences are well formed. And with the rise of large language models (LLMs), AI can even compose entire emails (or love poems) on our behalf. Video calls are becoming increasingly subjected to real-time filters, which not only allow us to alter the appearance of our surroundings—e.g., by showing a large library of books behind the speaker, signaling erudition—but are now beginning to alter our facial features and expressions. The anticipated rise of augmented reality will only accelerate these trends.
These developments, as well as more sci-fi-like imminent future developments, are altering human communication in fundamental ways. Much of our capacity for communication evolved, biologically and culturally, in order to solve issues of cooperation and coordination. We have no idea what happens when our ability to transmit and interpret facial, acoustic, and linguistic signals is completely AI-mediated. Can we trust others, without being able to look them in the eye because AI algorithms have altered their eye contact, or when AI has enhanced the honesty-signaling efficacy of their language? Or, more positively, can AI enhance cross-cultural communication by reducing the chance for misunderstanding? These are the kinds of questions we explore in this area of research.
Sample Project: Lie Detection (Ongoing)
Lie detection algorithms attract few users but vastly increase accusation rates.
People are not very good at detecting lies, which may explain why they refrain from accusing others of lying, given the social costs attached to false accusations—both for the accuser and the accused. In the algorithmic Lie Detection project, we consider how this social balance might be disrupted by the availability of lie-detection algorithms powered by artificial intelligence. Will people elect to use lie detection algorithms that perform better than humans and, if so, will they show less restraint in their accusations? We built a machine learning classifier whose accuracy (67%) was significantly better than human accuracy (50%) in a lie-detection task, and conducted an incentivized lie-detection experiment in which we measured participants’ propensity to use the algorithm, as well as the impact of that use on accusation rates (for an overview of the study design, see Figure 1).
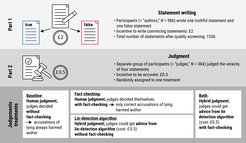
Figure 1. Overview of the study design. The study consisted of two parts. In Part 1, participants (= authors) wrote one true and one false statement; in Part 2, a separate sample of participants (= judges) judged four statements. Four different treatments existed. In the baseline treatment, participants decided by themselves, without fact-checking (= all accusations led to a reduction of payoffs for the author); in the fact-checking treatment, participants decided by themselves, with fact-checking (only accusations that were justified led to a reduction of payoffs for the author); in the lie-detection algorithm treatment, judges could purchase the advice from a lie-detection algorithm, but fact-checking was not present; in the both treatment, judges could purchase advice from a lie-detection algorithm.
Our results reveal that only a few people (33%) who elect to use the algorithm drastically increase their accusation rates (from 25% in the baseline condition up to 86% when the algorithm flags a statement as a lie). They make more false accusations (18pp increase), while the probability of a lie remaining undetected is much lower in this group (36pp decrease). We consider individual motivations for using lie detection algorithms and the social implications of these algorithms (see Figure 2 for results).
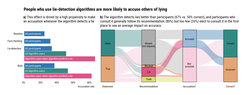
Figure 2. Main experimental results. (a) Accusation rate in each of the four treatments. In the lie-detection algorithm treatment and the both treatment (= where participants had access to our AI lie detection algorithm), accusation rates are also shown for the subset who elected to use the algorithm, as well as for the sub-subset of these participants whose lie-detection algorithm tagged the target statement as a lie. (b) Detailed stage-by-stage data for the lie-detection algorithm treatment and the both treatment, showing the AI’s guess (not requested, blocked, lie, truth) for false and true target statements, the subsequent decision of the participant depending on the algorithm’s guess, and the accuracy of this decision.
Key Reference
Sample Project: Blurry Face (Ongoing)
How do filters that blur people’s appearance affect prosocial behavior?
In the not-so-distant future, augmented reality (AR) glasses might become as ubiquitous as smartphones are today. The arguments in favor of this potential future: AR goggles allow us to receive information without having to look (down) at a phone, and they can—as the name suggests—augment our reality by applying real-time filters on our social environment.
One plausible use that has been popularized by multiple episodes of Black Mirror is the application of blurring filters that obfuscate disturbing events but can also depersonalize the appearance of (selective) other persons.
What if these applications hit the market, and people could choose which individuals or groups to blur out? How would it affect their prosociality and empathy toward them? On the flipside, could such filters help to make decisions in the interest of the greater good?
Not wanting to wait until such a technology is available (and potentially more difficult to regulate), we wanted to find out. We conducted three experiments (total N = 611). In each experiment, participants were randomly assigned to the blurry or original condition. In the blurry condition, the other person appeared, as the name suggests, blurred. Participants then first decided how to split an amount of money among themselves and the blurred recipient (also known as Dictator Game, see Figure 3, left pane). After that, they decided how to split money between the other participant and the World Food Program (also known as Charity Game, see Figure 3, right pane). For multiple rounds, participants decided on both tasks, which had real financial consequences as we paid out one of the rounds.
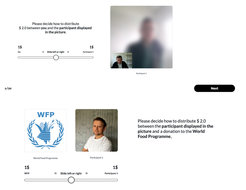
Figure 3. Left pane: Dictator Game with the blurred appearance of the recipient. Right pane: Charity Game with the recipient shown in a non-blurred original picture.
In Studies 1 and 2 we used static images, while in Study 3 we used a video conference. Instead of ten rounds, Study 3 entailed five rounds. Participants saw their partner’s face for 30 seconds. The partner’s facial appearance was either blurred or not.
The overall behavioral pattern across all three studies suggests that people reliably use de-personalization for selfish purposes but unreliably for pro-social purposes (see results of a mini meta-analysis of all three studies in Figure 4). For the Dictator Game, the results are always significant and go in the same direction across all three studies. However, the results for the Charity Game are inconsistent across the three studies. While, in theory, filters could be used for good or bad, bad use seems more likely than good use.

Figure 4. Results of a mini meta-analysis across all three studies show a consistent effect of blur filters increasing selfish behavior in the Dictator Game (left pane) and inconsistent findings for the Charity Game (right pane).
Sample Project: Minimal Turing Test (Ongoing)
Humans can overcome machine impostors and achieve communication even with minimal means.
Interactions between humans and bots are increasingly common online, and a pressing issue concerns the ability of humans to adequately discern whom they are interacting with. The Turing test is a classic thought experiment testing humans’ ability to distinguish a bot impostor from a real human by exchanging text messages (Turing, 1950, Computing Machinery and Intelligence). In the Minimal Turing Test project, we propose a version of the Turing test that avoids natural language. This allows us to study the foundations of human communication, as participants are forced to develop novel ways to signal their human identity even against bots that only copy human behavior faithfully. In particular, we investigate the relative roles of emerging conventions (i.e., repeating what has already proven successful before) and reciprocal interaction (i.e. interdependence between behaviors) in determining successful communication in a minimal environment.
Participants in our task could communicate only by moving an abstract shape in a 2D space (Figure 5). We asked them to assess their online social interaction as being with a human partner or a bot impostor. The main hypotheses were that access to the interaction history of a pair would make a bot impostor more deceptive and interrupt the formation of novel conventions between the human participants: Copying their previous interactions prevents humans from successfully communicating through repeating what already worked before. By comparing bots that imitate behavior from the same or a different dyad, we find that impostors are harder to detect when they copy the participants’ own partners (Figure 1), leading to less conventional interactions. We also show that reciprocity is beneficial for communicative success when the bot impostor prevents conventionality.
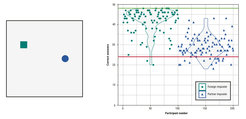
Image: MPI for Human Development
Adapted from Müller, Brinkmann, Winters, & Pescetelli (2023)
Original image licensed unter CC BY-NC 4.0
We conclude that machine impostors can avoid detection and interrupt the formation of stable conventions by imitating past interactions, and that both reciprocity and conventionality are adaptive communicative strategies under the right circumstances. Our results provide novel insights into the emergence of communication and suggest that online bots mining personal information, e.g., on social media, might become indistinguishable from humans more easily. However, even in this case, reciprocal interaction should remain a powerful mechanism for detecting bot impostors.
Key References