Formal Methods in Lifespan Psychology
Research Scientists
Andreas Brandmaier
Marie K. Deserno (until 11/2021)
Hannes Diemerling (as of 02/2023)
Charles C. Driver (until 02/2022)
Maike M. Kleemeyer (until 03/2021)
Ylva Köhncke (until 12/2022)
Ulman Lindenberger
Aaron Peikert
Timo von Oertzen (Associate Research Scientist)
Reliability in Cognitive Neuroscience
Modeling Longitudinal Data on Human Cognitive Development
Exploratory and Data-Driven Modeling Approaches
Since it was founded by the late Paul B. Baltes in 1981, the Center for Lifespan Psychology has sought to promote conceptual and methodological innovation within developmental psychology and in an interdisciplinary context. Over the years, the critical examination of relations among theory, method, and data has evolved into a distinct feature of the Center.
Based on the tenets of lifespan psychology, the Formal Methods project seeks to develop and refine statistical methods and research designs that articulate human development across different timescales, levels of analysis, and functional domains. Hence, the project is characterized by an emphasis on methodology, understood as the reciprocal interplay between concepts and methods that is at the heart of scientific progress. The project’s mission is to create a methodological foundation and an evolving statistical toolbox for high-quality research on lifespan development that allows researchers to address difficult problems rigorously and transparently.
During the reporting period, the project has focused on four domains of inquiry: (1) estimating the reliability of human brain imaging techniques; (2) modeling the dimensionality of age-related changes in cognition, as well as brain–cognition associations; (3) refining hypotheses through comprehensive exploratory data analysis; and (4) making scientific reports computationally reproducible.
Reliability in Cognitive Neuroscience
Cognitive neuroscience has paid relatively little attention to the psychometric properties of measures attained from structural and functional magnetic resonance imaging (MRI) protocols. In 2018, we introduced the intraclass effect decomposition (ICED) framework to overcome this shortcoming (Brandmaier et al., 2018). ICED allows researchers to quantify the effects of different measurement characteristics, such as day, session, or scanner, on measurement reliability. Using the ICED framework, we estimated the reliability of white-matter microstructure measurements using myelin water fraction (MWF) and an alternative estimation method (geomT2IEW), which are both meant to characterize the myelination of axons in the human brain. We found that reliability of regional microstructural characteristics in major white matter tracts is good to excellent (Anand et al., 2022). Next, we extended our model to accommodate hemispheric differences; we found that there were no significant differences in reliability between hemispheres. We then shifted our focus to the reliability of multi-parameter mapping, a quantitative MRI approach, which allows for quantitative estimates of various aspects of the human brain’s microanatomy. In a sample of healthy young adults, we found that reliabilities of between-person differences were high for all four quantitative parameters of interest when regarding whole-brain gray and white matter (Wenger et al., 2022). However, reliabilities varied greatly across different regions of the brain (see Figure 1 for whole-brain voxel-wise reliability maps). This finding has major implications for the interpretability of region-specific differences in brain–behavior relations.
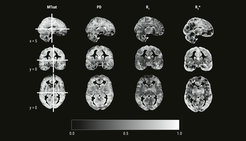
Image: MPI for Human Development
Adapted from Wenger et al. (2022)
Original image licensed under CC BY 4.0
Key References
Modeling Longitudinal Data on Human Cognitive Development
Together with colleagues from the Berlin Aging Studies and collaborators from the Lifebrain consortium (see Lifebrain website: https://www.lifebrain.uio.no/), project members participated in the multivariate modeling of longitudinal data on human cognitive development. For instance, we implemented a latent-variable approach to modeling region-specific gray-matter integrity in participants of the Berlin Aging Study II (Koehncke et al., 2021; see Berlin Aging Studies). Also, in a meta-analysis of about 500,000 participants, we explored the associations among measures of brain volume, cognition, and socioeconomic status, and found that the associations of socioeconomic status, brain, and cognition are heterogeneous across European and United States cohorts (Walhovd et al., 2022). Other examples for methodological contributions of project members to the Lifebrain consortium include the analysis of lifestyle-related risk factors and their cumulative associations with hippocampal and total gray matter volume across the adult lifespan (Binnewies et al., 2023), associations of depression and regional brain structure across the adult lifespan (Binnewies et al., 2022), change–change association between medial-temporal lobe integrity and episodic memory (Johanssen et al., 2022), and genetic predictors of working memory performance and in vivo dopamine integrity in aging (Karalija et al., 2021; see Berlin Aging Studies).
Key Reference
Exploratory and Data-Driven Modeling Approaches
Building models fully informed by theory is impossible when data sets are large and theoretical predictions are not available for all variables and their interrelations. In such instances, researchers may start with a core model guided by theory and then face the problem of which additional variables should be included. In earlier work, we have shown that Structural Equation Model (SEM) trees and forests provide a straightforward solution to this variable selection problem (Brandmaier et al., 2016), as they allow researchers to select predictors of parameter heterogeneity in multivariate models, and provide information on which variables might be missing from their models and, by implication, from the theories on which these models are based.
However, SEM trees and forests are computationally demanding, and it has sometimes proven infeasible to fit larger models on standard computers. As a remedy, we have proposed to guide the construction of SEM trees by score-based tests (Arnold et al., 2021). In comparison to the originally proposed SEM tree algorithm, these tests are faster to compute by orders of magnitude, have higher statistical power, and do not suffer from variable selection bias. In subsequent work, we have shown how score-based tests can be used to identify predictors of individual differences in SEM when the predictors and the parameters of interest are linearly associated (individual parameter contribution regression; see Arnold et al., 2020, 2021).
Key References
Reproducibility
A data analysis is reproducible if identical results can be obtained from the same statistical analysis and the same data. Reproducibility is a requirement for transparent science and, importantly, facilitates replication of reported findings by peer scientists, that is, the attempt to obtain novel but consistent results with the same statistical analysis on new data. We proposed a unified workflow to achieve reproducibility of both statistical analyses and the accompanying scientific reports. This workflow helps researchers adopt Open Science principles in their research, of which reproducibility is a cornerstone. To that end, the workflow leverages tools from software engineering that have proven to be useful for collaboration, transparency, automation, and reproducibility (Peikert et al., 2021; Peikert & Brandmaier, 2021). Additional work has focused on integrating reproducibility and pre-registration in a common, conceptually grounded framework (Dissertation Aaron Peikert). According to this framework, pre-registration's main benefit is reducing the uncertainty of evidential support of a given study for a given theory. We argue that combining reproducibility with pre-registration fosters scientific progress by enhancing efficiency and transparency.
Key Reference